Open Access
ARTICLE
Leveraging Multimodal Ensemble Fusion-Based Deep Learning for COVID-19 on Chest Radiographs
1 Department of Medical Equipment Technology, College of Applied Medical Sciences, Majmaah University, Al Majmaah, 11952, Saudi Arabia
2 Department of Biomedical Engineering, Bannari Amman Institute of Technology, Sathyamangalam, 638401, India
3 Department of Computer Science and Engineering, K.Ramakrishnan College of Engineering, Tiruchirappalli, 621112, India
4 Institute of Biomedical Engineering, Saveetha School of Engineering, Saveetha Institute of Medical and Technical Sciences, Saveetha University, Chennai, 602105, India
5 Department of Applied Data Science, Noroff University College, Kristiansand, Norway
6 Artificial Intelligence Research Center (AIRC), College of Engineering and Information Technology, Ajman University, Ajman, United Arab Emirates
7 Department of Electrical and Computer Engineering, Lebanese American University, Byblos, Lebanon
8 Department of Software, Kongju National University, Cheonan, 31080, Korea
9 Division of Computer Engineering, Hansung University, Seoul, 02876, Korea
* Corresponding Author: Mohamed Yacin Sikkandar. Email:
Computer Systems Science and Engineering 2023, 47(1), 873-889. https://doi.org/10.32604/csse.2023.035730
Received 01 September 2022; Accepted 21 December 2022; Issue published 26 May 2023
Abstract
Recently, COVID-19 has posed a challenging threat to researchers, scientists, healthcare professionals, and administrations over the globe, from its diagnosis to its treatment. The researchers are making persistent efforts to derive probable solutions for managing the pandemic in their areas. One of the widespread and effective ways to detect COVID-19 is to utilize radiological images comprising X-rays and computed tomography (CT) scans. At the same time, the recent advances in machine learning (ML) and deep learning (DL) models show promising results in medical imaging. Particularly, the convolutional neural network (CNN) model can be applied to identifying abnormalities on chest radiographs. While the epidemic of COVID-19, much research is led on processing the data compared with DL techniques, particularly CNN. This study develops an improved fruit fly optimization with a deep learning-enabled fusion (IFFO-DLEF) model for COVID-19 detection and classification. The major intention of the IFFO-DLEF model is to investigate the presence or absence of COVID-19. To do so, the presented IFFO-DLEF model applies image pre-processing at the initial stage. In addition, the ensemble of three DL models such as DenseNet169, EfficientNet, and ResNet50, are used for feature extraction. Moreover, the IFFO algorithm with a multilayer perceptron (MLP) classification model is utilized to identify and classify COVID-19. The parameter optimization of the MLP approach utilizing the IFFO technique helps in accomplishing enhanced classification performance. The experimental result analysis of the IFFO-DLEF model carried out on the CXR image database portrayed the better performance of the presented IFFO-DLEF model over recent approaches.Keywords
Cite This Article
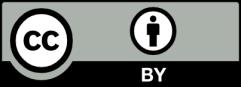
This work is licensed under a Creative Commons Attribution 4.0 International License , which permits unrestricted use, distribution, and reproduction in any medium, provided the original work is properly cited.