Open Access
ARTICLE
Tight Sandstone Image Augmentation for Image Identification Using Deep Learning
School of Computer & Information Technology, Northeast Petroleum University, Daqing, 163318, China
* Corresponding Author: Chunsheng Li. Email:
Computer Systems Science and Engineering 2023, 47(1), 1209-1231. https://doi.org/10.32604/csse.2023.034395
Received 15 July 2022; Accepted 08 October 2022; Issue published 26 May 2023
Abstract
Intelligent identification of sandstone slice images using deep learning technology is the development trend of mineral identification, and accurate mineral particle segmentation is the most critical step for intelligent identification. A typical identification model requires many training samples to learn as many distinguishable features as possible. However, limited by the difficulty of data acquisition, the high cost of labeling, and privacy protection, this has led to a sparse sample number and cannot meet the training requirements of deep learning image identification models. In order to increase the number of samples and improve the training effect of deep learning models, this paper proposes a tight sandstone image data augmentation method by combining the advantages of the data deformation method and the data oversampling method in the Putaohua reservoir in the Sanzhao Sag of the Songliao Basin as the target area. First, the Style Generative Adversarial Network (StyleGAN) is improved to generate high-resolution tight sandstone images to improve data diversity. Second, we improve the Automatic Data Augmentation (AutoAugment) algorithm to search for the optimal augmentation strategy to expand the data scale. Finally, we design comparison experiments to demonstrate that this method has obvious advantages in generating image quality and improving the identification effect of deep learning models in real application scenarios.Keywords
Cite This Article
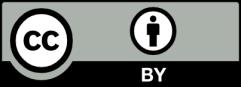
This work is licensed under a Creative Commons Attribution 4.0 International License , which permits unrestricted use, distribution, and reproduction in any medium, provided the original work is properly cited.