Open Access
ARTICLE
Statistical Data Mining with Slime Mould Optimization for Intelligent Rainfall Classification
1 Department of Mathematics, Vignan’s Institute of Information Technology, Visakhapatnam, 530049, India
2 Department of Computer Science and Engineering, University Institute of Engineering and Technology (UIET),Guru Nanak University, Hyderabad, India
3 Department of Electrical Engineering, College of Engineering, Jouf University, Saudi Arabia
4 Department of Computer Science and Engineering, GITAM School of Technology, Vishakhapatnam Campus, GITAM (Deemed to be a University), Vishakhapatnam, India
5 Department of Applied Data Science, Noroff University College, Kristiansand, Norway
6 Artificial Intelligence Research Center (AIRC), College of Engineering and Information Technology, Ajman University, Ajman, United Arab Emirates
7 Department of Electrical and Computer Engineering, Lebanese American University, Byblos, Lebanon
8 Department of Software, Kongju National University, Cheonan, 31080, Korea
9 Division of Computer Engineering, Hansung University, Seoul, 02876, Korea
* Corresponding Author: Seifedine Kadry. Email:
Computer Systems Science and Engineering 2023, 47(1), 919-935. https://doi.org/10.32604/csse.2023.034213
Received 09 July 2022; Accepted 10 March 2023; Issue published 26 May 2023
Abstract
Statistics are most crucial than ever due to the accessibility of huge counts of data from several domains such as finance, medicine, science, engineering, and so on. Statistical data mining (SDM) is an interdisciplinary domain that examines huge existing databases to discover patterns and connections from the data. It varies in classical statistics on the size of datasets and on the detail that the data could not primarily be gathered based on some experimental strategy but conversely for other resolves. Thus, this paper introduces an effective statistical Data Mining for Intelligent Rainfall Prediction using Slime Mould Optimization with Deep Learning (SDMIRP-SMODL) model. In the presented SDMIRP-SMODL model, the feature subset selection process is performed by the SMO algorithm, which in turn minimizes the computation complexity. For rainfall prediction. Convolution neural network with long short-term memory (CNN-LSTM) technique is exploited. At last, this study involves the pelican optimization algorithm (POA) as a hyperparameter optimizer. The experimental evaluation of the SDMIRP-SMODL approach is tested utilizing a rainfall dataset comprising 23682 samples in the negative class and 1865 samples in the positive class. The comparative outcomes reported the supremacy of the SDMIRP-SMODL model compared to existing techniques.Keywords
Cite This Article
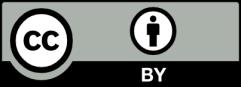