Open Access
ARTICLE
CBOE Volatility Index Forecasting under COVID-19: An Integrated BiLSTM-ARIMA-GARCH Model
1 Department of Applied Artificial Intelligence, Sungkyunkwan University, Seoul, 03063, Korea
2 Department of Human-Artificial Intelligence Interaction, Sungkyunkwan University, Seoul, 03063, Korea
3 Department of Interaction Science, Sungkyunkwan University, Seoul, 03063, Korea
* Corresponding Author: Jang Hyun Kim. Email:
Computer Systems Science and Engineering 2023, 47(1), 121-134. https://doi.org/10.32604/csse.2023.033247
Received 12 June 2022; Accepted 29 January 2023; Issue published 26 May 2023
Abstract
After the outbreak of COVID-19, the global economy entered a deep freeze. This observation is supported by the Volatility Index (VIX), which reflects the market risk expected by investors. In the current study, we predicted the VIX using variables obtained from the sentiment analysis of data on Twitter posts related to the keyword “COVID-19,” using a model integrating the bidirectional long-term memory (BiLSTM), autoregressive integrated moving average (ARIMA) algorithm, and generalized autoregressive conditional heteroskedasticity (GARCH) model. The Linguistic Inquiry and Word Count (LIWC) program and Valence Aware Dictionary for Sentiment Reasoning (VADER) model were utilized as sentiment analysis methods. The results revealed that during COVID-19, the proposed integrated model, which trained both the Twitter sentiment values and historical VIX values, presented better results in forecasting the VIX in time-series regression and direction prediction than those of the other existing models.Keywords
Cite This Article
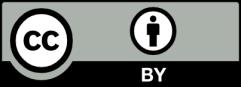
This work is licensed under a Creative Commons Attribution 4.0 International License , which permits unrestricted use, distribution, and reproduction in any medium, provided the original work is properly cited.