Open Access
ARTICLE
Intelligent Intrusion Detection for Industrial Internet of Things Using Clustering Techniques
College of Computing and Information Technology, University of Tabuk, Tabuk, 71491, Saudi Arabia
* Corresponding Author: Ahamed Aljuhani. Email:
Computer Systems Science and Engineering 2023, 46(3), 2899-2915. https://doi.org/10.32604/csse.2023.036657
Received 08 October 2022; Accepted 28 December 2022; Issue published 03 April 2023
Abstract
The rapid growth of the Internet of Things (IoT) in the industrial sector has given rise to a new term: the Industrial Internet of Things (IIoT). The IIoT is a collection of devices, apps, and services that connect physical and virtual worlds to create smart, cost-effective, and scalable systems. Although the IIoT has been implemented and incorporated into a wide range of industrial control systems, maintaining its security and privacy remains a significant concern. In the IIoT contexts, an intrusion detection system (IDS) can be an effective security solution for ensuring data confidentiality, integrity, and availability. In this paper, we propose an intelligent intrusion detection technique that uses principal components analysis (PCA) as a feature engineering method to choose the most significant features, minimize data dimensionality, and enhance detection performance. In the classification phase, we use clustering algorithms such as K-medoids and K-means to determine whether a given flow of IIoT traffic is normal or attack for binary classification and identify the group of cyberattacks according to its specific type for multi-class classification. To validate the effectiveness and robustness of our proposed model, we validate the detection method on a new driven IIoT dataset called X-IIoTID. The performance results showed our proposed detection model obtained a higher accuracy rate of 99.79% and reduced error rate of 0.21% when compared to existing techniques.Keywords
Cite This Article
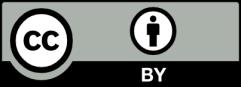
This work is licensed under a Creative Commons Attribution 4.0 International License , which permits unrestricted use, distribution, and reproduction in any medium, provided the original work is properly cited.