Open Access
ARTICLE
Feature Selection with Deep Reinforcement Learning for Intrusion Detection System
1
Department of Computer Science and Engineering, SRM Institute of Science and Technology, Ramapuram, Chennai, 600089, India
2
Department of Computing Technologies, SRM Institute of Science and Technology, Kattankulathur, Chennai, 603203, India
* Corresponding Author: S. Priya. Email:
Computer Systems Science and Engineering 2023, 46(3), 3339-3353. https://doi.org/10.32604/csse.2023.030630
Received 30 March 2022; Accepted 12 May 2022; Issue published 03 April 2023
Abstract
An intrusion detection system (IDS) becomes an important tool for ensuring security in the network. In recent times, machine learning (ML) and deep learning (DL) models can be applied for the identification of intrusions over the network effectively. To resolve the security issues, this paper presents a new Binary Butterfly Optimization algorithm based on Feature Selection with DRL technique, called BBOFS-DRL for intrusion detection. The proposed BBOFSDRL model mainly accomplishes the recognition of intrusions in the network. To attain this, the BBOFS-DRL model initially designs the BBOFS algorithm based on the traditional butterfly optimization algorithm (BOA) to elect feature subsets. Besides, DRL model is employed for the proper identification and classification of intrusions that exist in the network. Furthermore, beetle antenna search (BAS) technique is applied to tune the DRL parameters for enhanced intrusion detection efficiency. For ensuring the superior intrusion detection outcomes of the BBOFS-DRL model, a wide-ranging experimental analysis is performed against benchmark dataset. The simulation results reported the supremacy of the BBOFS-DRL model over its recent state of art approaches.Keywords
Cite This Article
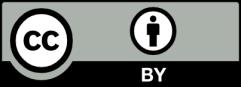
This work is licensed under a Creative Commons Attribution 4.0 International License , which permits unrestricted use, distribution, and reproduction in any medium, provided the original work is properly cited.