Open Access
ARTICLE
Enhanced Image Captioning Using Features Concatenation and Efficient Pre-Trained Word Embedding
1 Department of Data Science, Faculty of Artificial Intelligence, Kafrelsheikh University, Kafrelsheikh, 33511, Egypt
2 Department of Electrical Engineering, Faculty of Engineering, Kafrelsheikh University, Kafrelsheikh, 33511, Egypt
3 Department of Computer Science, Faculty of Computer and Information Science, Mansoura University, Mansoura, 35516, Egypt
* Corresponding Author: Samar Elbedwehy. Email:
Computer Systems Science and Engineering 2023, 46(3), 3637-3652. https://doi.org/10.32604/csse.2023.038376
Received 10 December 2022; Accepted 02 February 2023; Issue published 03 April 2023
Abstract
One of the issues in Computer Vision is the automatic development of descriptions for images, sometimes known as image captioning. Deep Learning techniques have made significant progress in this area. The typical architecture of image captioning systems consists mainly of an image feature extractor subsystem followed by a caption generation lingual subsystem. This paper aims to find optimized models for these two subsystems. For the image feature extraction subsystem, the research tested eight different concatenations of pairs of vision models to get among them the most expressive extracted feature vector of the image. For the caption generation lingual subsystem, this paper tested three different pre-trained language embedding models: Glove (Global Vectors for Word Representation), BERT (Bidirectional Encoder Representations from Transformers), and TaCL (Token-aware Contrastive Learning), to select from them the most accurate pre-trained language embedding model. Our experiments showed that building an image captioning system that uses a concatenation of the two Transformer based models SWIN (Shifted window) and PVT (Pyramid Vision Transformer) as an image feature extractor, combined with the TaCL language embedding model is the best result among the other combinations.Keywords
Cite This Article
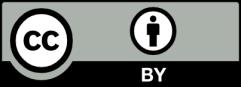
This work is licensed under a Creative Commons Attribution 4.0 International License , which permits unrestricted use, distribution, and reproduction in any medium, provided the original work is properly cited.