Open Access
ARTICLE
Multi-Strategy Boosted Spider Monkey Optimization Algorithm for Feature Selection
Glorious Sun School of Business and Management, Donghua University, Shanghai, 200051, China
* Corresponding Author: Shuilin Chen. Email:
Computer Systems Science and Engineering 2023, 46(3), 3619-3635. https://doi.org/10.32604/csse.2023.038025
Received 24 November 2022; Accepted 02 February 2023; Issue published 03 April 2023
Abstract
To solve the problem of slow convergence and easy to get into the local optimum of the spider monkey optimization algorithm, this paper presents a new algorithm based on multi-strategy (ISMO). First, the initial population is generated by a refracted opposition-based learning strategy to enhance diversity and ergodicity. Second, this paper introduces a non-linear adaptive dynamic weight factor to improve convergence efficiency. Then, using the crisscross strategy, using the horizontal crossover to enhance the global search and vertical crossover to keep the diversity of the population to avoid being trapped in the local optimum. At last, we adopt a Gauss-Cauchy mutation strategy to improve the stability of the algorithm by mutation of the optimal individuals. Therefore, the application of ISMO is validated by ten benchmark functions and feature selection. It is proved that the proposed method can resolve the problem of feature selection.Keywords
Cite This Article
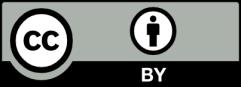
This work is licensed under a Creative Commons Attribution 4.0 International License , which permits unrestricted use, distribution, and reproduction in any medium, provided the original work is properly cited.