Open Access
ARTICLE
Network Intrusion Detection in Internet of Blended Environment Using Ensemble of Heterogeneous Autoencoders (E-HAE)
1 ISAA Lab., Department of AI Convergence Network, Ajou University, Suwon, 16499, Korea
2 ISAA Lab., Institute for Information and Communication, Ajou University, Suwon, 16499, Korea
3 Department of Computer Science and Information Technology, Massey University, Auckland, 0745, New Zealand
4 Department of Cyber Security, Ajou University, Suwon, 16499, Korea
* Corresponding Author: Jin Kwak. Email:
Computer Systems Science and Engineering 2023, 46(3), 3261-3284. https://doi.org/10.32604/csse.2023.037615
Received 10 November 2022; Accepted 09 February 2023; Issue published 03 April 2023
Abstract
Contemporary attackers, mainly motivated by financial gain, consistently devise sophisticated penetration techniques to access important information or data. The growing use of Internet of Things (IoT) technology in the contemporary convergence environment to connect to corporate networks and cloud-based applications only worsens this situation, as it facilitates multiple new attack vectors to emerge effortlessly. As such, existing intrusion detection systems suffer from performance degradation mainly because of insufficient considerations and poorly modeled detection systems. To address this problem, we designed a blended threat detection approach, considering the possible impact and dimensionality of new attack surfaces due to the aforementioned convergence. We collectively refer to the convergence of different technology sectors as the internet of blended environment. The proposed approach encompasses an ensemble of heterogeneous probabilistic autoencoders that leverage the corresponding advantages of a convolutional variational autoencoder and long short-term memory variational autoencoder. An extensive experimental analysis conducted on the TON_IoT dataset demonstrated 96.02% detection accuracy. Furthermore, performance of the proposed approach was compared with various single model (autoencoder)-based network intrusion detection approaches: autoencoder, variational autoencoder, convolutional variational autoencoder, and long short-term memory variational autoencoder. The proposed model outperformed all compared models, demonstrating F1-score improvements of 4.99%, 2.25%, 1.92%, and 3.69%, respectively.Keywords
Cite This Article
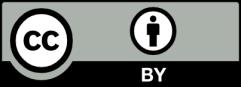