Open Access
ARTICLE
TC-Net: A Modest & Lightweight Emotion Recognition System Using Temporal Convolution Network
1 Sejong University Software Convergence Department, Seoul, 05006, Korea
2 Mohamed Bin Zayed University of Artificial Intelligence (MBZUAI), Abu Dhabi, 3838-111188, United Arab Emirates
* Corresponding Author: Soonil Kwon. Email:
Computer Systems Science and Engineering 2023, 46(3), 3355-3369. https://doi.org/10.32604/csse.2023.037373
Received 01 November 2022; Accepted 09 February 2023; Issue published 03 April 2023
Abstract
Speech signals play an essential role in communication and provide an efficient way to exchange information between humans and machines. Speech Emotion Recognition (SER) is one of the critical sources for human evaluation, which is applicable in many real-world applications such as healthcare, call centers, robotics, safety, and virtual reality. This work developed a novel TCN-based emotion recognition system using speech signals through a spatial-temporal convolution network to recognize the speaker’s emotional state. The authors designed a Temporal Convolutional Network (TCN) core block to recognize long-term dependencies in speech signals and then feed these temporal cues to a dense network to fuse the spatial features and recognize global information for final classification. The proposed network extracts valid sequential cues automatically from speech signals, which performed better than state-of-the-art (SOTA) and traditional machine learning algorithms. Results of the proposed method show a high recognition rate compared with SOTA methods. The final unweighted accuracy of 80.84%, and 92.31%, for interactive emotional dyadic motion captures (IEMOCAP) and berlin emotional dataset (EMO-DB), indicate the robustness and efficiency of the designed model.Keywords
Cite This Article
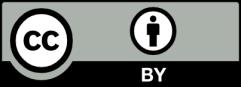
This work is licensed under a Creative Commons Attribution 4.0 International License , which permits unrestricted use, distribution, and reproduction in any medium, provided the original work is properly cited.