Open Access
ARTICLE
An Efficient and Robust Hand Gesture Recognition System of Sign Language Employing Finetuned Inception-V3 and Efficientnet-B0 Network
1 Department of Computer Science, Islamia College University, Peshawar, 25120, Pakistan
2 Department of Computer Science and Engineering, Chung-Ang University, Seoul, 06974, Korea
3 Department of Computer Engineering, Cyprus International University, Lefkosa, 99010, Rep. of North Cyprus
4 College of Art and Technology, Chung-Ang.University, Anseong, 17546, Korea
* Corresponding Author: Sanghyun Seo. Email:
Computer Systems Science and Engineering 2023, 46(3), 3509-3525. https://doi.org/10.32604/csse.2023.037258
Received 28 October 2022; Accepted 13 January 2023; Issue published 03 April 2023
Abstract
Hand Gesture Recognition (HGR) is a promising research area with an extensive range of applications, such as surgery, video game techniques, and sign language translation, where sign language is a complicated structured form of hand gestures. The fundamental building blocks of structured expressions in sign language are the arrangement of the fingers, the orientation of the hand, and the hand’s position concerning the body. The importance of HGR has increased due to the increasing number of touchless applications and the rapid growth of the hearing-impaired population. Therefore, real-time HGR is one of the most effective interaction methods between computers and humans. Developing a user-free interface with good recognition performance should be the goal of real-time HGR systems. Nowadays, Convolutional Neural Network (CNN) shows great recognition rates for different image-level classification tasks. It is challenging to train deep CNN networks like VGG-16, VGG-19, Inception-v3, and Efficientnet-B0 from scratch because only some significant labeled image datasets are available for static hand gesture images. However, an efficient and robust hand gesture recognition system of sign language employing finetuned Inception-v3 and Efficientnet-Bo network is proposed to identify hand gestures using a comparative small HGR dataset. Experiments show that Inception-v3 achieved 90% accuracy and 0.93% precision, 0.91% recall, and 0.90% f1-score, respectively, while EfficientNet-B0 achieved 99% accuracy and 0.98%, 0.97%, 0.98%, precision, recall, and f1-score respectively.Keywords
Cite This Article
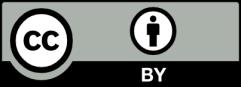
This work is licensed under a Creative Commons Attribution 4.0 International License , which permits unrestricted use, distribution, and reproduction in any medium, provided the original work is properly cited.