Open Access
ARTICLE
Real-Time Multi-Feature Approximation Model-Based Efficient Brain Tumor Classification Using Deep Learning Convolution Neural Network Model
1 Department of Computer Science and Engineering, School of Computing, SRM Institute of Science and Technology, Kattankulathur, Chengalpattu, Chennai, 603203, Tamilnadu, India
2 Department of Information Technology, MLR Institute of Technology, Hyderabad, 500043, Telangana, India
3 Department of Computing Technologies, School of Computing, SRM Institute of Science and Technology, Kattankulathur, Chengalpattu, Chennai, 603203, Tamilnadu, India
* Corresponding Author: M. Baskar. Email:
Computer Systems Science and Engineering 2023, 46(3), 3883-3899. https://doi.org/10.32604/csse.2023.037050
Received 21 October 2022; Accepted 24 February 2023; Issue published 03 April 2023
Abstract
The deep learning models are identified as having a significant impact on various problems. The same can be adapted to the problem of brain tumor classification. However, several deep learning models are presented earlier, but they need better classification accuracy. An efficient Multi-Feature Approximation Based Convolution Neural Network (CNN) model (MFA-CNN) is proposed to handle this issue. The method reads the input 3D Magnetic Resonance Imaging (MRI) images and applies Gabor filters at multiple levels. The noise-removed image has been equalized for its quality by using histogram equalization. Further, the features like white mass, grey mass, texture, and shape are extracted from the images. Extracted features are trained with deep learning Convolution Neural Network (CNN). The network has been designed with a single convolution layer towards dimensionality reduction. The texture features obtained from the brain image have been transformed into a multi-dimensional feature matrix, which has been transformed into a single-dimensional feature vector at the convolution layer. The neurons of the intermediate layer are designed to measure White Mass Texture Support (WMTS), Gray Mass Texture Support (GMTS), White Mass Covariance Support (WMCS), Gray Mass Covariance Support (GMCS), and Class Texture Adhesive Support (CTAS). In the test phase, the neurons at the intermediate layer compute the support as mentioned above values towards various classes of images. Based on that, the method adds a Multi-Variate Feature Similarity Measure (MVFSM). Based on the importance of MVFSM, the process finds the class of brain image given and produces an efficient result.Keywords
Cite This Article
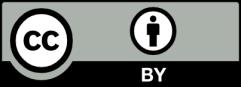