Open Access
ARTICLE
Adaptive Learning Video Streaming with QoE in Multi-Home Heterogeneous Networks
1 Department of Electronics and Communication, Sona College of Technology, Salem, 636005, Tamil Nadu, India
2 Department of Information Technology, K. S. R. College of Engineering, Tiruchengode, 637215, Tamil Nadu, India
* Corresponding Author: S. Vijayashaarathi. Email:
Computer Systems Science and Engineering 2023, 46(3), 2881-2897. https://doi.org/10.32604/csse.2023.036864
Received 14 October 2022; Accepted 21 December 2022; Issue published 03 April 2023
Abstract
In recent years, real-time video streaming has grown in popularity. The growing popularity of the Internet of Things (IoT) and other wireless heterogeneous networks mandates that network resources be carefully apportioned among versatile users in order to achieve the best Quality of Experience (QoE) and performance objectives. Most researchers focused on Forward Error Correction (FEC) techniques when attempting to strike a balance between QoE and performance. However, as network capacity increases, the performance degrades, impacting the live visual experience. Recently, Deep Learning (DL) algorithms have been successfully integrated with FEC to stream videos across multiple heterogeneous networks. But these algorithms need to be changed to make the experience better without sacrificing packet loss and delay time. To address the previous challenge, this paper proposes a novel intelligent algorithm that streams video in multi-home heterogeneous networks based on network-centric characteristics. The proposed framework contains modules such as Intelligent Content Extraction Module (ICEM), Channel Status Monitor (CSM), and Adaptive FEC (AFEC). This framework adopts the Cognitive Learning-based Scheduling (CLS) Module, which works on the deep Reinforced Gated Recurrent Networks (RGRN) principle and embeds them along with the FEC to achieve better performances. The complete framework was developed using the Objective Modular Network Testbed in C++ (OMNET++), Internet networking (INET), and Python 3.10, with Keras as the front end and Tensorflow 2.10 as the back end. With extensive experimentation, the proposed model outperforms the other existing intelligent models in terms of improving the QoE, minimizing the End-to-End Delay (EED), and maintaining the highest accuracy (98%) and a lower Root Mean Square Error (RMSE) value of 0.001.Keywords
Cite This Article
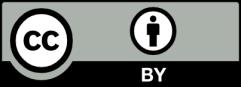