Open Access
ARTICLE
A Novel Computationally Efficient Approach to Identify Visually Interpretable Medical Conditions from 2D Skeletal Data
1 Tiger Analytics, Chennai, 600041, India
2 College of Engineering Guindy, Anna University, Chennai, 600025, India
* Corresponding Author: Praveen Jesudhas. Email:
Computer Systems Science and Engineering 2023, 46(3), 2995-3015. https://doi.org/10.32604/csse.2023.036778
Received 12 October 2022; Accepted 28 December 2022; Issue published 03 April 2023
Abstract
Timely identification and treatment of medical conditions could facilitate faster recovery and better health. Existing systems address this issue using custom-built sensors, which are invasive and difficult to generalize. A low-complexity scalable process is proposed to detect and identify medical conditions from 2D skeletal movements on video feed data. Minimal set of features relevant to distinguish medical conditions: AMF, PVF and GDF are derived from skeletal data on sampled frames across the entire action. The AMF (angular motion features) are derived to capture the angular motion of limbs during a specific action. The relative position of joints is represented by PVF (positional variation features). GDF (global displacement features) identifies the direction of overall skeletal movement. The discriminative capability of these features is illustrated by their variance across time for different actions. The classification of medical conditions is approached in two stages. In the first stage, a low-complexity binary LSTM classifier is trained to distinguish visual medical conditions from general human actions. As part of stage 2, a multi-class LSTM classifier is trained to identify the exact medical condition from a given set of visually interpretable medical conditions. The proposed features are extracted from the 2D skeletal data of NTU RGB + D and then used to train the binary and multi-class LSTM classifiers. The binary and multi-class classifiers observed average F1 scores of 77% and 73%, respectively, while the overall system produced an average F1 score of 69% and a weighted average F1 score of 80%. The multi-class classifier is found to utilize 10 to 100 times fewer parameters than existing 2D CNN-based models while producing similar levels of accuracy.Keywords
Cite This Article
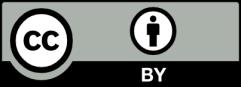
This work is licensed under a Creative Commons Attribution 4.0 International License , which permits unrestricted use, distribution, and reproduction in any medium, provided the original work is properly cited.