Open Access
ARTICLE
A Novel Cluster Analysis-Based Crop Dataset Recommendation Method in Precision Farming
1 Department of Computer Science & Engineering, Bapuji Institute of Engineering & Technology, Davangere, Karnataka, India
2 Department of Information Technology, Faculty of Computing and Information Technology, King Abdulaziz University, Jeddah, Saudi Arabia
3 Department of Information Science & Engineering, Bapuji Institute of Engineering & Technology, Davangere, Karnataka, India
4 Department of Electrical Engineering, Faculty of Engineering at Rabigh, King Abdulaziz University, Jeddah, Saudi Arabia
5 Department of Electrical Engineering, College of Engineering, Northern Border University, Arar, Saudi Arabia
* Corresponding Author: B. R. Sreenivasa. Email:
Computer Systems Science and Engineering 2023, 46(3), 3239-3260. https://doi.org/10.32604/csse.2023.036629
Received 07 October 2022; Accepted 09 February 2023; Issue published 03 April 2023
Abstract
Data mining and analytics involve inspecting and modeling large pre-existing datasets to discover decision-making information. Precision agriculture uses data mining to advance agricultural developments. Many farmers aren’t getting the most out of their land because they don’t use precision agriculture. They harvest crops without a well-planned recommendation system. Future crop production is calculated by combining environmental conditions and management behavior, yielding numerical and categorical data. Most existing research still needs to address data preprocessing and crop categorization/classification. Furthermore, statistical analysis receives less attention, despite producing more accurate and valid results. The study was conducted on a dataset about Karnataka state, India, with crops of eight parameters taken into account, namely the minimum amount of fertilizers required, such as nitrogen, phosphorus, potassium, and pH values. The research considers rainfall, season, soil type, and temperature parameters to provide precise cultivation recommendations for high productivity. The presented algorithm converts discrete numerals to factors first, then reduces levels. Second, the algorithm generates six datasets, two from Case-1 (dataset with many numeric variables), two from Case-2 (dataset with many categorical variables), and one from Case-3 (dataset with reduced factor variables). Finally, the algorithm outputs a class membership allocation based on an extended version of the K-means partitioning method with lambda estimation. The presented work produces mixed-type datasets with precisely categorized crops by organizing data based on environmental conditions, soil nutrients, and geo-location. Finally, the prepared dataset solves the classification problem, leading to a model evaluation that selects the best dataset for precise crop prediction.Keywords
Cite This Article
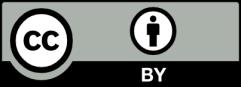
This work is licensed under a Creative Commons Attribution 4.0 International License , which permits unrestricted use, distribution, and reproduction in any medium, provided the original work is properly cited.