Open Access
ARTICLE
Optimal Deep Learning Based Intruder Identification in Industrial Internet of Things Environment
1 Department of Computer Science, College of Science and Arts, Sharurah, Najran University, Saudi Arabia
2 Department of Information Systems, College of Computer and Information Sciences, Princess Nourah bint Abdulrahman University, P.O. Box 84428, Riyadh, 11671, Saudi Arabia
3 Department of Industrial Engineering, College of Engineering at Alqunfudah, Umm Al-Qura University, Saudi Arabia
4 Department of Computer Science, Faculty of Computers and Information Technology, Future University in Egypt, New Cairo, 11835, Egypt
5 Computer Department, Applied College, Najran University, Najran, 66462, Saudi Arabia
6 Department of Computer Science, College of Computer Engineering and Sciences, Prince Sattam bin Abdulaziz University, Al-Kharj, 16273, Saudi Arabia
* Corresponding Author: Mesfer Al Duhayyim. Email:
Computer Systems Science and Engineering 2023, 46(3), 3121-3139. https://doi.org/10.32604/csse.2023.036352
Received 27 September 2022; Accepted 25 November 2022; Issue published 03 April 2023
Abstract
With the increased advancements of smart industries, cybersecurity has become a vital growth factor in the success of industrial transformation. The Industrial Internet of Things (IIoT) or Industry 4.0 has revolutionized the concepts of manufacturing and production altogether. In industry 4.0, powerful Intrusion Detection Systems (IDS) play a significant role in ensuring network security. Though various intrusion detection techniques have been developed so far, it is challenging to protect the intricate data of networks. This is because conventional Machine Learning (ML) approaches are inadequate and insufficient to address the demands of dynamic IIoT networks. Further, the existing Deep Learning (DL) can be employed to identify anonymous intrusions. Therefore, the current study proposes a Hunger Games Search Optimization with Deep Learning-Driven Intrusion Detection (HGSODL-ID) model for the IIoT environment. The presented HGSODL-ID model exploits the linear normalization approach to transform the input data into a useful format. The HGSO algorithm is employed for Feature Selection (HGSO-FS) to reduce the curse of dimensionality. Moreover, Sparrow Search Optimization (SSO) is utilized with a Graph Convolutional Network (GCN) to classify and identify intrusions in the network. Finally, the SSO technique is exploited to fine-tune the hyper-parameters involved in the GCN model. The proposed HGSODL-ID model was experimentally validated using a benchmark dataset, and the results confirmed the superiority of the proposed HGSODL-ID method over recent approaches.Keywords
Cite This Article
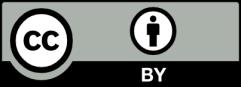
This work is licensed under a Creative Commons Attribution 4.0 International License , which permits unrestricted use, distribution, and reproduction in any medium, provided the original work is properly cited.