Open Access
ARTICLE
SMOGN, MFO, and XGBoost Based Excitation Current Prediction Model for Synchronous Machine
1 Department of Mechanical Engineering, National Chung Cheng University, Chiayi, 62102, Taiwan
2 Advanced Institute of Manufacturing with High-Tech Innovations (AIM-HI), National Chung Cheng University, Chiayi, 62102, Taiwan
* Corresponding Author: Her-Terng Yau. Email:
Computer Systems Science and Engineering 2023, 46(3), 2687-2709. https://doi.org/10.32604/csse.2023.036293
Received 24 September 2022; Accepted 08 December 2022; Issue published 03 April 2023
Abstract
The power factor is the ratio between the active and apparent power, and it is available to determine the operational capability of the intended circuit or the parts. The excitation current of the synchronous motor is an essential parameter required for adjusting the power factor because it determines whether the motor is under the optimal operating status. Although the excitation current should predict with the experimental devices, such a method is unsuitable for online real-time prediction. The artificial intelligence algorithm can compensate for the defect of conventional measurement methods requiring the measuring devices and the model optimization is compared during the research process. In this article, the load current, power factor, and power factor errors available in the existing dataset are used as the input parameters for training the proposed artificial intelligence algorithms to select the optimal algorithm according to the training result, for this algorithm to have higher accuracy. The SMOGN (Synthetic Minority Over-Sampling Technique for Regression with Gaussian Noise) is selected for the research by which the data and the MFO (Moth-flame optimization algorithm) are created for the model to adjust and optimize the parameters automatically. In addition to enhancing the prediction accuracy for the excitation current, the automatic parameter adjusting method also allows the researchers not specializing in the professional algorithm to apply such application method more efficiently. The final result indicated that the prediction accuracy has reached “Mean Absolute Error (MAE) = 0.0057, Root Mean Square Error (RMSE) = 0.0093 and R2 score = 0.9973”. Applying this method to the motor control would be much easier for the power factor adjustment in the future because it allows the motor to operate under the optimal power status to reduce energy consumption while enhancing working efficiency.Keywords
Cite This Article
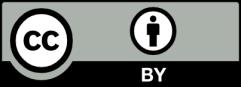
This work is licensed under a Creative Commons Attribution 4.0 International License , which permits unrestricted use, distribution, and reproduction in any medium, provided the original work is properly cited.