Open Access
ARTICLE
Question-Answering Pair Matching Based on Question Classification and Ensemble Sentence Embedding
1 Department of Computer Science and Engineering, Seoul National University of Science and Technology, Seoul, 01811, Korea
2 Department of Industrial Engineering/Graduate School of Data Science, Seoul National University of Science and Technology, Seoul, 01811, Korea
* Corresponding Author: Hyuk-Yoon Kwon. Email:
Computer Systems Science and Engineering 2023, 46(3), 3471-3489. https://doi.org/10.32604/csse.2023.035570
Received 26 August 2022; Accepted 03 November 2022; Issue published 03 April 2023
Abstract
Question-answering (QA) models find answers to a given question. The necessity of automatically finding answers is increasing because it is very important and challenging from the large-scale QA data sets. In this paper, we deal with the QA pair matching approach in QA models, which finds the most relevant question and its recommended answer for a given question. Existing studies for the approach performed on the entire dataset or datasets within a category that the question writer manually specifies. In contrast, we aim to automatically find the category to which the question belongs by employing the text classification model and to find the answer corresponding to the question within the category. Due to the text classification model, we can effectively reduce the search space for finding the answers to a given question. Therefore, the proposed model improves the accuracy of the QA matching model and significantly reduces the model inference time. Furthermore, to improve the performance of finding similar sentences in each category, we present an ensemble embedding model for sentences, improving the performance compared to the individual embedding models. Using real-world QA data sets, we evaluate the performance of the proposed QA matching model. As a result, the accuracy of our final ensemble embedding model based on the text classification model is 81.18%, which outperforms the existing models by 9.81%∼14.16% point. Moreover, in terms of the model inference speed, our model is faster than the existing models by 2.61∼5.07 times due to the effective reduction of search spaces by the text classification model.Keywords
Cite This Article
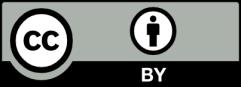
This work is licensed under a Creative Commons Attribution 4.0 International License , which permits unrestricted use, distribution, and reproduction in any medium, provided the original work is properly cited.