Open Access
ARTICLE
Covid-19 Detection Using Deep Correlation-Grey Wolf Optimizer
1 Department of Computer Science and Engineering, Karpagam College of Engineering, Coimbatore, 641032, India
2 Department of Computer Engineering, Lebanese French University, Erbil, 44001, Iraq
3 Department of Computer Science, College of Computers and Information Technology, Taif University, P. O. Box 11099, Taif, 21944, Saudi Arabia
4 Department of Information Technology, College of Computer and Information Technology, Taif University, PO Box 11099, Taif, 21994, Saudi Arabia
5 Department of Mathematics, Faculty of Science, Mansoura University, Mansoura, 35516, Egypt
6 Department of Computational Mathematics, Science, and Engineering (CMSE), Michigan State University, East Lansing, 48824, MI, USA
* Corresponding Author: Mohamed Abouhawwash. Email:
Computer Systems Science and Engineering 2023, 46(3), 2933-2945. https://doi.org/10.32604/csse.2023.034288
Received 13 July 2022; Accepted 28 December 2022; Issue published 03 April 2023
A correction of this article was approved in:
Correction: Covid-19 Detection Using Deep Correlation-Grey Wolf Optimizer
Read correction
Abstract
The immediate and quick spread of the coronavirus has become a life-threatening disease around the globe. The widespread illness has dramatically changed almost all sectors, moving from offline to online, resulting in a new normal lifestyle for people. The impact of coronavirus is tremendous in the healthcare sector, which has experienced a decline in the first quarter of 2020. This pandemic has created an urge to use computer-aided diagnosis techniques for classifying the Covid-19 dataset to reduce the burden of clinical results. The current situation motivated me to choose correlation-based development called correlation-based grey wolf optimizer to perform accurate classification. A proposed multistage model helps to identify Covid from Computed Tomography (CT) scan image. The first process uses a convolutional neural network (CNN) for extracting the feature from the CT scans. The Pearson coefficient filter method is applied to remove redundant and irrelevant features. Finally, the Grey wolf optimizer is used to choose optimal features. Experimental analysis proves that this determines the optimal characteristics to detect the deadly disease. The proposed model’s accuracy is 14% higher than the krill herd and bacterial foraging optimization for severe accurate respiratory syndrome image (SARS-CoV-2 CT) dataset. The COVID CT image dataset is 22% higher than the existing krill herd and bacterial foraging optimization techniques. The proposed techniques help to increase the classification accuracy of the algorithm in most cases, which marks the stability of the stated result. Comparative analysis reveals that the proposed classification technique to predict COVID-19 with maximum accuracy of 98% outperforms other competitive approaches.Keywords
Cite This Article
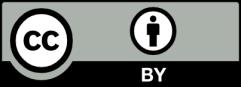
This work is licensed under a Creative Commons Attribution 4.0 International License , which permits unrestricted use, distribution, and reproduction in any medium, provided the original work is properly cited.