Open Access
ARTICLE
Enhancing the Adversarial Transferability with Channel Decomposition
1 Sichuan Normal University, Chengdu, 610066, China
2 Jinan Geotechnical Investigation and Surveying Institute, Jinan, 250000, China
3 School of Computer Science and Engineering, Sichuan University of Science & Engineering, Zigong, 643000, China
4 School of Computer Science, Southwest Petroleum University, Chengdu, 610500, China
5 AECC Sichuan Gas Turbine Estab, Mianyang, 621000, China
6 School of Physics, University of Electronic Science and Technology of China, Chengdu, 610056, China
7 School of Power and Energy, Northwestern Polytechnical University, Xi’an, 710072, China
8 Department of Chemistry, Physics and Atmospheric Science, Jackson State University, Jackson, MS, USA
* Corresponding Author: Wenli Zeng. Email:
Computer Systems Science and Engineering 2023, 46(3), 3075-3085. https://doi.org/10.32604/csse.2023.034268
Received 12 July 2022; Accepted 13 November 2022; Issue published 03 April 2023
Abstract
The current adversarial attacks against deep learning models have achieved incredible success in the white-box scenario. However, they often exhibit weak transferability in the black-box scenario, especially when attacking those with defense mechanisms. In this work, we propose a new transfer-based black-box attack called the channel decomposition attack method (CDAM). It can attack multiple black-box models by enhancing the transferability of the adversarial examples. On the one hand, it tunes the gradient and stabilizes the update direction by decomposing the channels of the input example and calculating the aggregate gradient. On the other hand, it helps to escape from local optima by initializing the data point with random noise. Besides, it could combine with other transfer-based attacks flexibly. Extensive experiments on the standard ImageNet dataset show that our method could significantly improve the transferability of adversarial attacks. Compared with the state-of-the-art method, our approach improves the average success rate from 88.2% to 96.6% when attacking three adversarially trained black-box models, demonstrating the remaining shortcomings of existing deep learning models.Keywords
Cite This Article
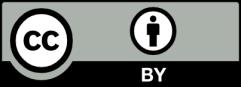
This work is licensed under a Creative Commons Attribution 4.0 International License , which permits unrestricted use, distribution, and reproduction in any medium, provided the original work is properly cited.