Open Access
ARTICLE
Deep Learning Algorithm for Detection of Protein Remote Homology
1 Kahramanmaras Sutcu Imam University, Kahramanmaras, 46100, Turkey
2 Adana Alparslan Turkes Science and Technology University, Adana, 01250, Turkey
3 Çukurova University, Adana, 01330, Turkey
* Corresponding Author: Fahriye Gemci. Email:
Computer Systems Science and Engineering 2023, 46(3), 3703-3713. https://doi.org/10.32604/csse.2023.032706
Received 26 May 2022; Accepted 12 July 2022; Issue published 03 April 2023
Abstract
The study aims to find a successful solution by using computer algorithms to detect remote homologous proteins, which is a significant problem in the bioinformatics field. In this experimental study, structural classification of proteins (SCOP) 1.53, SCOP benchmark, and the newly created SCOP protein database from the structural classification of proteins—extended (SCOPe) 2.07 were used to detect remote homolog proteins. N-gram method and then Term Frequency-Inverse Document Frequency (TF-IDF) weighting were performed to extract features of the protein sequences taken from these databases. Next, a smoothing process on the obtained features was performed to avoid misclassification. Finally, the proteins with balanced features were classified into remote homologs using the built deep learning architecture. As a result, remote homologous proteins have been detected with novel deep learning architecture using both negative and positive protein instances with a mean accuracy of 89.13% and a mean relative operating characteristic (ROC) score of 88.39%. This experiment demonstrated the following: 1) The successful outcome of this study in detecting remote homology is auspicious in discovering new proteins and thus in drug discovery in medicine. 2) Natural language processing (NLP) techniques were used successfully in bioinformatics, 3) the importance of choosing the correct n-value in the n-gram process, 4) the necessity of using not only positive but negative instances in a classification problem, and 5) how effective the processes, such as smoothing, are in the classification accuracy in an imbalanced dataset. 6) The deep learning architecture gives better results than the support vector machine (SVM) model on the smoothed data to detect proteins’ remote homology.Keywords
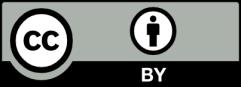