Open Access
ARTICLE
Anomaly Detection and Classification in Streaming PMU Data in Smart Grids
1 Department of Computational Intelligence, SRM Institute of Science and Technology, Chennai, 603203, India
2 Department of Electrical and Electronics Engineering, SRM Institute of Science and Technology, Chennai, 603203, India
3 Department of Computer Science and Engineering, Government College of Engineering, Salem, 636011, India
* Corresponding Author: R. Annie Uthra. Email:
Computer Systems Science and Engineering 2023, 46(3), 3387-3401. https://doi.org/10.32604/csse.2023.029904
Received 14 March 2022; Accepted 13 June 2022; Issue published 03 April 2023
Abstract
The invention of Phasor Measurement Units (PMUs) produce synchronized phasor measurements with high resolution real time monitoring and control of power system in smart grids that make possible. PMUs are used in transmitting data to Phasor Data Concentrators (PDC) placed in control centers for monitoring purpose. A primary concern of system operators in control centers is maintaining safe and efficient operation of the power grid. This can be achieved by continuous monitoring of the PMU data that contains both normal and abnormal data. The normal data indicates the normal behavior of the grid whereas the abnormal data indicates fault or abnormal conditions in power grid. As a result, detecting anomalies/abnormal conditions in the fast flowing PMU data that reflects the status of the power system is critical. A novel methodology for detecting and categorizing abnormalities in streaming PMU data is presented in this paper. The proposed method consists of three modules namely, offline Gaussian Mixture Model (GMM), online GMM for identifying anomalies and clustering ensemble model for classifying the anomalies. The significant features of the proposed method are detecting anomalies while taking into account of multivariate nature of the PMU dataset, adapting to concept drift in the flowing PMU data without retraining the existing model unnecessarily and classifying the anomalies. The proposed model is implemented in Python and the testing results prove that the proposed model is well suited for detection and classification of anomalies on the fly.Keywords
Cite This Article
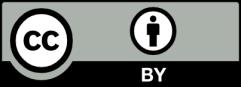
This work is licensed under a Creative Commons Attribution 4.0 International License , which permits unrestricted use, distribution, and reproduction in any medium, provided the original work is properly cited.