Open Access
ARTICLE
An Efficient Indoor Localization Based on Deep Attention Learning Model
1 Department of Computer Science, College of Science and Arts, Jouf University, Qurayyat, Saudi Arabia
2 Information System Department, Faculty of Computers and Information, Assiut University, Egypt
3 Computer Science Department, Faculty of Computers and Information, Assiut University, Assiut, Egypt
4 Department of Computer Science, Faculty of Computers and Information, South Valley University, Qena, Egypt
* Corresponding Author: Amr Abozeid. Email:
Computer Systems Science and Engineering 2023, 46(2), 2637-2650. https://doi.org/10.32604/csse.2023.037761
Received 16 November 2022; Accepted 06 January 2023; Issue published 09 February 2023
Abstract
Indoor localization methods can help many sectors, such as healthcare centers, smart homes, museums, warehouses, and retail malls, improve their service areas. As a result, it is crucial to look for low-cost methods that can provide exact localization in indoor locations. In this context, image-based localization methods can play an important role in estimating both the position and the orientation of cameras regarding an object. Image-based localization faces many issues, such as image scale and rotation variance. Also, image-based localization’s accuracy and speed (latency) are two critical factors. This paper proposes an efficient 6-DoF deep-learning model for image-based localization. This model incorporates the channel attention module and the Scale Pyramid Module (SPM). It not only enhances accuracy but also ensures the model’s real-time performance. In complex scenes, a channel attention module is employed to distinguish between the textures of the foregrounds and backgrounds. Our model adapted an SPM, a feature pyramid module for dealing with image scale and rotation variance issues. Furthermore, the proposed model employs two regressions (two fully connected layers), one for position and the other for orientation, which increases outcome accuracy. Experiments on standard indoor and outdoor datasets show that the proposed model has a significantly lower Mean Squared Error (MSE) for both position and orientation. On the indoor 7-Scenes dataset, the MSE for the position is reduced to 0.19 m and 6.25° for the orientation. Furthermore, on the outdoor Cambridge landmarks dataset, the MSE for the position is reduced to 0.63 m and 2.03° for the orientation. According to the findings, the proposed approach is superior and more successful than the baseline methods.Keywords
Cite This Article
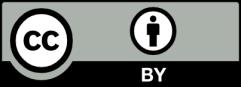
This work is licensed under a Creative Commons Attribution 4.0 International License , which permits unrestricted use, distribution, and reproduction in any medium, provided the original work is properly cited.