Open Access
ARTICLE
A General Linguistic Steganalysis Framework Using Multi-Task Learning
1 School of Computer and Communication Engineering, Changsha University of Science and Technology, Changsha, 410114, China
2 School of Computer Science and Electronic Engineering, University of Essex, Colchester, CO4 3SQ, UK
3 School of Computer Science, Hunan University of Technology and Business, Changsha, 410205, China
* Corresponding Author: Lingyun Xiang. Email:
Computer Systems Science and Engineering 2023, 46(2), 2383-2399. https://doi.org/10.32604/csse.2023.037067
Received 21 October 2022; Accepted 21 December 2022; Issue published 09 February 2023
Abstract
Prevailing linguistic steganalysis approaches focus on learning sensitive features to distinguish a particular category of steganographic texts from non-steganographic texts, by performing binary classification. While it remains an unsolved problem and poses a significant threat to the security of cyberspace when various categories of non-steganographic or steganographic texts coexist. In this paper, we propose a general linguistic steganalysis framework named LS-MTL, which introduces the idea of multi-task learning to deal with the classification of various categories of steganographic and non-steganographic texts. LS-MTL captures sensitive linguistic features from multiple related linguistic steganalysis tasks and can concurrently handle diverse tasks with a constructed model. In the proposed framework, convolutional neural networks (CNNs) are utilized as private base models to extract sensitive features for each steganalysis task. Besides, a shared CNN is built to capture potential interaction information and share linguistic features among all tasks. Finally, LS-MTL incorporates the private and shared sensitive features to identify the detected text as steganographic or non-steganographic. Experimental results demonstrate that the proposed framework LS-MTL outperforms the baseline in the multi-category linguistic steganalysis task, while average Acc, Pre, and Rec are increased by 0.5%, 1.4%, and 0.4%, respectively. More ablation experimental results show that LS-MTL with the shared module has robust generalization capability and achieves good detection performance even in the case of spare data.Keywords
Cite This Article
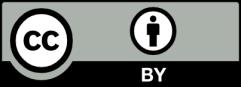
This work is licensed under a Creative Commons Attribution 4.0 International License , which permits unrestricted use, distribution, and reproduction in any medium, provided the original work is properly cited.