Open Access
ARTICLE
Modeling of Sensor Enabled Irrigation Management for Intelligent Agriculture Using Hybrid Deep Belief Network
1 Department of Software Engineering, College of Computer Science and Engineering, University of Jeddah, Jeddah, Saudi Arabia
2 King Abdul Aziz City for Science and Technology, Riyadh, Kingdom of Saudi Arabia
3 Aditya Engineering College Affiliated to JNTUK (Kakinada), Surampalem, India
4 Department of MBA, Vignan’s Institute of Information and Technology (A), Duvvada, Visakhapatnam, AP, 530049, India
5 School of Electrical and Electronic Engineering, Engineering Campus, Universiti Sains Malaysia (USM), Nibong Tebal, Penang, 14300, Malaysia
6 Department of Information Systems, College of Computer and Information Sciences, Princess Nourah bint Abdulrahman University, Riyadh, 11564, Saudi Arabia
7 SISAu Research Group, Universidad Tecnológica Indoamérica, Ambato, 180103, Ecuador
8 Faculty of Computers and Information, South Valley University, Qena, 83523, Egypt
* Corresponding Author: José Varela-Aldás. Email:
Computer Systems Science and Engineering 2023, 46(2), 2319-2335. https://doi.org/10.32604/csse.2023.036721
Received 10 October 2022; Accepted 14 December 2022; Issue published 09 February 2023
Abstract
Artificial intelligence (AI) technologies and sensors have recently received significant interest in intellectual agriculture. Accelerating the application of AI technologies and agriculture sensors in intellectual agriculture is urgently required for the growth of modern agriculture and will help promote smart agriculture. Automatic irrigation scheduling systems were highly required in the agricultural field due to their capability to manage and save water deficit irrigation techniques. Automatic learning systems devise an alternative to conventional irrigation management through the automatic elaboration of predictions related to the learning of an agronomist. With this motivation, this study develops a modified black widow optimization with a deep belief network-based smart irrigation system (MBWODBN-SIS) for intelligent agriculture. The MBWODBN-SIS algorithm primarily enables the Internet of Things (IoT) based sensors to collect data forwarded to the cloud server for examination purposes. Besides, the MBWODBN-SIS technique applies the deep belief network (DBN) model for different types of irrigation classification: average, high needed, highly not needed, and not needed. The MBWO algorithm is used for the hyperparameter tuning process. A wide-ranging experiment was conducted, and the comparison study stated the enhanced outcomes of the MBWODBN-SIS approach to other DL models with maximum accuracy of 95.73%.Keywords
Cite This Article
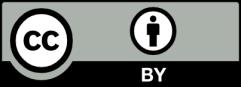
This work is licensed under a Creative Commons Attribution 4.0 International License , which permits unrestricted use, distribution, and reproduction in any medium, provided the original work is properly cited.