Open Access
ARTICLE
Data Analytics on Unpredictable Pregnancy Data Records Using Ensemble Neuro-Fuzzy Techniques
1 Faculty of Information and Communication Engineering, Anna University, Chennai, 600025, India
2 Department of Computer Science and Engineering, KSR College of Engineering, Tiruchengode, 637215, India
* Corresponding Author: C. Vairavel. Email:
Computer Systems Science and Engineering 2023, 46(2), 2159-2175. https://doi.org/10.32604/csse.2023.036598
Received 06 October 2022; Accepted 08 December 2022; Issue published 09 February 2023
Abstract
The immune system goes through a profound transformation during pregnancy, and certain unexpected maternal complications have been correlated to this transition. The ability to correctly examine, diagnoses, and predict pregnancy-hastened diseases via the available big data is a delicate problem since the range of information continuously increases and is scalable. Many approaches for disease diagnosis/classification have been established with the use of data mining concepts. However, such methods do not provide an appropriate classification/diagnosis model. Furthermore, single learning approaches are used to create the bulk of these systems. Classification issues may be made more accurate by combining predictions from many different techniques. As a result, we used the Ensembling of Neuro-Fuzzy (E-NF) method to perform a high-level classification of medical diseases. E-NF is a layered computational model with self-learning and self-adaptive capabilities to deal with specific problems, such as the handling of imprecise and ambiguous data that may lead to uncertainty concerns that specifically emerge during the classification stage. Preprocessing data, Training phase, Ensemble phase, and Testing phase make up the complete procedure for the suggested task. Data preprocessing includes feature extraction and dimensionality reduction. Besides such processes, the training phase includes the fuzzification process of medical data. Moreover, training of input data was done using four types of NF techniques: Fuzzy Adaptive Learning Control Network (FALCON), Adaptive Network-based Fuzzy Inference System (ANFIS), Self Constructing Neural Fuzzy Inference Network (SONFIN) and/Evolving Fuzzy Neural Network (EFuNN). Later, in the ensemble phase, all the NF methods’ predicted outcomes are integrated, and finally, the test results are evaluated in the testing phase. The outcomes indicate that the method could predict impaired glucose tolerance, preeclampsia, gestational hypertensive abnormalities, bacteriuria, and iron deficiency anaemia better than the others. In addition, the model exposed the capability to be utilized as an autonomous learning strategy, specifically in the early stages of pregnancy, examinations, and clinical guidelines for disease interventions.Keywords
Cite This Article
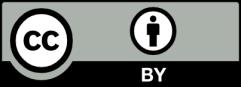