Open Access
ARTICLE
Improved Siamese Palmprint Authentication Using Pre-Trained VGG16-Palmprint and Element-Wise Absolute Difference
College of Computers and Information Sciences, Jouf University, Sakaka, 72314, Saudi Arabia
* Corresponding Author: Ayman Mohamed Mostafa. Email:
Computer Systems Science and Engineering 2023, 46(2), 2299-2317. https://doi.org/10.32604/csse.2023.036567
Received 05 October 2022; Accepted 21 December 2022; Issue published 09 February 2023
Abstract
Palmprint identification has been conducted over the last two decades in many biometric systems. High-dimensional data with many uncorrelated and duplicated features remains difficult due to several computational complexity issues. This paper presents an interactive authentication approach based on deep learning and feature selection that supports Palmprint authentication. The proposed model has two stages of learning; the first stage is to transfer pre-trained VGG-16 of ImageNet to specific features based on the extraction model. The second stage involves the VGG-16 Palmprint feature extraction in the Siamese network to learn Palmprint similarity. The proposed model achieves robust and reliable end-to-end Palmprint authentication by extracting the convolutional features using VGG-16 Palmprint and the similarity of two input Palmprint using the Siamese network. The second stage uses the CASIA dataset to train and test the Siamese network. The suggested model outperforms comparable studies based on the deep learning approach achieving accuracy and EER of 91.8% and 0.082%, respectively, on the CASIA left-hand images and accuracy and EER of 91.7% and 0.084, respectively, on the CASIA right-hand images.Keywords
Cite This Article
M. Ezz, W. Alanazi, A. M. Mostafa, E. Hamouda, M. K. Elbashir et al., "Improved siamese palmprint authentication using pre-trained vgg16-palmprint and element-wise absolute difference," Computer Systems Science and Engineering, vol. 46, no.2, pp. 2299–2317, 2023. https://doi.org/10.32604/csse.2023.036567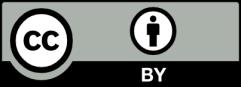