Open Access
ARTICLE
Augmenting Android Malware Using Conditional Variational Autoencoder for the Malware Family Classification
Soongsil University, Seoul, 06978, Korea
* Corresponding Author: Haehyun Cho. Email:
Computer Systems Science and Engineering 2023, 46(2), 2215-2230. https://doi.org/10.32604/csse.2023.036555
Received 04 October 2022; Accepted 23 November 2022; Issue published 09 February 2023
Abstract
Android malware has evolved in various forms such as adware that continuously exposes advertisements, banking malware designed to access users’ online banking accounts, and Short Message Service (SMS) malware that uses a Command & Control (C&C) server to send malicious SMS, intercept SMS, and steal data. By using many malicious strategies, the number of malware is steadily increasing. Increasing Android malware threats numerous users, and thus, it is necessary to detect malware quickly and accurately. Each malware has distinguishable characteristics based on its actions. Therefore, security researchers have tried to categorize malware based on their behaviors by conducting the familial analysis which can help analysists to reduce the time and cost for analyzing malware. However, those studies algorithms typically used imbalanced, well-labeled open-source dataset, and thus, it is very difficult to classify some malware families which only have a few number of malware. To overcome this challenge, previous data augmentation studies augmented data by visualizing malicious codes and used them for malware analysis. However, visualization of malware can result in misclassifications because the behavior information of the malware could be compromised. In this study, we propose an android malware familial analysis system based on a data augmentation method that preserves malware behaviors to create an effective multi-class classifier for malware family analysis. To this end, we analyze malware and use Application Programming Interface (APIs) and permissions that can reflect the behavior of malware as features. By using these features, we augment malware dataset to enable effective malware detection while preserving original malicious behaviors. Our evaluation results demonstrate that, when a model is created by using only the augmented data, a macro-F1 score of 0.65 and accuracy of 0.63%. On the other hand, when the augmented data and original malware are used together, the evaluation results show that a macro-F1 score of 0.91 and an accuracy of 0.99%.Keywords
Cite This Article
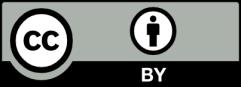