Open Access
ARTICLE
Using Recurrent Neural Network Structure and Multi-Head Attention with Convolution for Fraudulent Phone Text Recognition
School of Information and Electrical Engineering, Shandong Jianzhu University, Jinan, 250000, China
* Corresponding Author: Hongkui Xu. Email:
Computer Systems Science and Engineering 2023, 46(2), 2277-2297. https://doi.org/10.32604/csse.2023.036419
Received 29 September 2022; Accepted 14 December 2022; Issue published 09 February 2023
Abstract
Fraud cases have been a risk in society and people’s property security has been greatly threatened. In recent studies, many promising algorithms have been developed for social media offensive text recognition as well as sentiment analysis. These algorithms are also suitable for fraudulent phone text recognition. Compared to these tasks, the semantics of fraudulent words are more complex and more difficult to distinguish. Recurrent Neural Networks (RNN), the variants of RNN, Convolutional Neural Networks (CNN), and hybrid neural networks to extract text features are used by most text classification research. However, a single network or a simple network combination cannot obtain rich characteristic knowledge of fraudulent phone texts relatively. Therefore, a new model is proposed in this paper. In the fraudulent phone text, the knowledge that can be learned by the model includes the sequence structure of sentences, the correlation between words, the correlation of contextual semantics, the feature of keywords in sentences, etc. The new model combines a bidirectional Long-Short Term Memory Neural Network (BiLSTM) or a bidirectional Gate Recurrent United (BiGRU) and a Multi-Head attention mechanism module with convolution. A normalization layer is added after the output of the final hidden layer. BiLSTM or BiGRU is used to build the encoding and decoding layer. Multi-head attention mechanism module with convolution (MHAC) enhances the ability of the model to learn global interaction information and multi-granularity local interaction information in fraudulent sentences. A fraudulent phone text dataset is produced by us in this paper. The THUCNews data sets and fraudulent phone text data sets are used in experiments. Experiment results show that compared with the baseline model, the proposed model (LMHACL) has the best experiment results in terms of Accuracy, Precision, Recall, and F1 score on the two data sets. And the performance indexes on fraudulent phone text data sets are all above 0.94.Keywords
Cite This Article
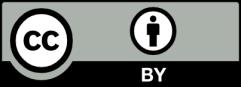