Open Access
ARTICLE
Earthworm Optimization with Improved SqueezeNet Enabled Facial Expression Recognition Model
1 Computer Science and Engineering Department, Gayatri Vidya Parishad College of Engineering for Women, Visakhapatnam, Andhra Pradesh, India
2 Department of Software Engineering, College of Computer Science and Engineering, University of Jeddah, Jeddah, Saudi Arabia
3 King Abdul Aziz City for Science and Technology, Riyadh, Kingdom of Saudi Arabia
4 Department of Computer Science and Engineering, Vignan’s Institute of Information Technology, Visakhapatnam, 530049, India
5 School of Electrical and Electronic Engineering, Engineering Campus, Universiti Sains Malaysia (USM), Nibong Tebal, Penang, 14300, Malaysia
6 Department of Information Systems, College of Computer and Information Sciences, Princess Nourah bint Abdulrahman University, Saudi Arabia
7 Department of Computer Science, University of Central Asia, Naryn, 722600, Kyrgyzstan
8 Faculty of Computers and Information, South Valley University, Qena, 83523, Egypt
* Corresponding Author: Hend Khalid Alkahtani. Email:
Computer Systems Science and Engineering 2023, 46(2), 2247-2262. https://doi.org/10.32604/csse.2023.036377
Received 28 September 2022; Accepted 08 December 2022; Issue published 09 February 2023
Abstract
Facial expression recognition (FER) remains a hot research area among computer vision researchers and still becomes a challenge because of high intra-class variations. Conventional techniques for this problem depend on hand-crafted features, namely, LBP, SIFT, and HOG, along with that a classifier trained on a database of videos or images. Many execute perform well on image datasets captured in a controlled condition; however not perform well in the more challenging dataset, which has partial faces and image variation. Recently, many studies presented an endwise structure for facial expression recognition by utilizing DL methods. Therefore, this study develops an earthworm optimization with an improved SqueezeNet-based FER (EWOISN-FER) model. The presented EWOISN-FER model primarily applies the contrast-limited adaptive histogram equalization (CLAHE) technique as a pre-processing step. In addition, the improved SqueezeNet model is exploited to derive an optimal set of feature vectors, and the hyperparameter tuning process is performed by the stochastic gradient boosting (SGB) model. Finally, EWO with sparse autoencoder (SAE) is employed for the FER process, and the EWO algorithm appropriately chooses the SAE parameters. A wide-ranging experimental analysis is carried out to examine the performance of the proposed model. The experimental outcomes indicate the supremacy of the presented EWOISN-FER technique.Keywords
Cite This Article
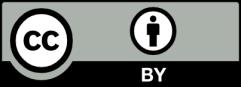
This work is licensed under a Creative Commons Attribution 4.0 International License , which permits unrestricted use, distribution, and reproduction in any medium, provided the original work is properly cited.