Open Access
ARTICLE
Identification of Key Links in Electric Power Operation Based-Spatiotemporal Mixing Convolution Neural Network
1 School of Electrical and Automation, Wuhan University, Wuhan, Hubei, 430072, China
2 Tus-Institute for Renewable Energy, Qinghai University, Xining, Qinghai, 810016, China
3 Electrical Engineering Department, Faculty of Engineering, Minia University, Minia, 61519, Egypt
* Corresponding Author: Bo Wang. Email:
Computer Systems Science and Engineering 2023, 46(2), 1487-1501. https://doi.org/10.32604/csse.2023.035377
Received 18 August 2022; Accepted 28 October 2022; Issue published 09 February 2023
Abstract
As the scale of the power system continues to expand, the environment for power operations becomes more and more complex. Existing risk management and control methods for power operations can only set the same risk detection standard and conduct the risk detection for any scenario indiscriminately. Therefore, more reliable and accurate security control methods are urgently needed. In order to improve the accuracy and reliability of the operation risk management and control method, this paper proposes a method for identifying the key links in the whole process of electric power operation based on the spatiotemporal hybrid convolutional neural network. To provide early warning and control of targeted risks, first, the video stream is framed adaptively according to the pixel changes in the video stream. Then, the optimized MobileNet is used to extract the feature map of the video stream, which contains both time-series and static spatial scene information. The feature maps are combined and non-linearly mapped to realize the identification of dynamic operating scenes. Finally, training samples and test samples are produced by using the whole process image of a power company in Xinjiang as a case study, and the proposed algorithm is compared with the unimproved MobileNet. The experimental results demonstrated that the method proposed in this paper can accurately identify the type and start and end time of each operation link in the whole process of electric power operation, and has good real-time performance. The average accuracy of the algorithm can reach 87.8%, and the frame rate is 61 frames/s, which is of great significance for improving the reliability and accuracy of security control methods.Keywords
Cite This Article
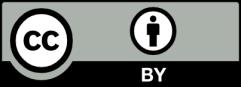
This work is licensed under a Creative Commons Attribution 4.0 International License , which permits unrestricted use, distribution, and reproduction in any medium, provided the original work is properly cited.