Open Access
ARTICLE
Computer-Aided Diagnosis for Tuberculosis Classification with Water Strider Optimization Algorithm
1 Department of Computational Science and Electronic, Universidad de la Costa, CUC, Barranquilla, 080002, Colombia
2 Biomedical Engineering Program, Universidad Simón Bolívar, Barranquilla, 080002, Colombia
3 Mechatronics Engineering Program, Universidad Simón Bolívar, Barranquilla, 080002, Colombia
4 Department of Medicine, Universidad del Norte, Barranquilla, 081007, Colombia
5 Department of Mathematics, Faculty of Science, New Valley University, El-Kharga, 72511, Egypt
* Corresponding Author: José Escorcia-Gutierrez. Email:
Computer Systems Science and Engineering 2023, 46(2), 1337-1353. https://doi.org/10.32604/csse.2023.035253
Received 13 August 2022; Accepted 28 October 2022; Issue published 09 February 2023
Abstract
Computer-aided diagnosis (CAD) models exploit artificial intelligence (AI) for chest X-ray (CXR) examination to identify the presence of tuberculosis (TB) and can improve the feasibility and performance of CXR for TB screening and triage. At the same time, CXR interpretation is a time-consuming and subjective process. Furthermore, high resemblance among the radiological patterns of TB and other lung diseases can result in misdiagnosis. Therefore, computer-aided diagnosis (CAD) models using machine learning (ML) and deep learning (DL) can be designed for screening TB accurately. With this motivation, this article develops a Water Strider Optimization with Deep Transfer Learning Enabled Tuberculosis Classification (WSODTL-TBC) model on Chest X-rays (CXR). The presented WSODTL-TBC model aims to detect and classify TB on CXR images. Primarily, the WSODTL-TBC model undergoes image filtering techniques to discard the noise content and U-Net-based image segmentation. Besides, a pre-trained residual network with a two-dimensional convolutional neural network (2D-CNN) model is applied to extract feature vectors. In addition, the WSO algorithm with long short-term memory (LSTM) model was employed for identifying and classifying TB, where the WSO algorithm is applied as a hyperparameter optimizer of the LSTM methodology, showing the novelty of the work. The performance validation of the presented WSODTL-TBC model is carried out on the benchmark dataset, and the outcomes were investigated in many aspects. The experimental development pointed out the betterment of the WSODTL-TBC model over existing algorithms.Keywords
Cite This Article
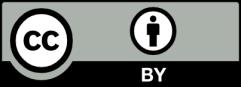
This work is licensed under a Creative Commons Attribution 4.0 International License , which permits unrestricted use, distribution, and reproduction in any medium, provided the original work is properly cited.