Open Access
ARTICLE
Enhanced Adaptive Brain-Computer Interface Approach for Intelligent Assistance to Disabled Peoples
1 Department of Computer Science, COMSATS University Islamabad, Sahiwal Campus, Sahiwal, 57000, Pakistan
2 Department of Information Systems, College of Computer Science and Information Systems, Najran University, Najran, 61441, Saudi Arabia
3 Anatomy Department, Medicine College, Najran University, Najran, 61441, Saudi Arabia
4 Computer Science Department, College of Computer Science and Information Systems, Najran University, Najran, 61441, Saudi Arabia
5 Radiological Sciences Department, College of Applied Medical Sciences and Information Systems, Najran University, Najran, 61441, Kingdom of Saudi Arabia
6 Electrical Engineering Department, College of Engineering, Najran University, Najran, 61441, Saudi Arabia
* Corresponding Author: Samar Alqhtani. Email:
Computer Systems Science and Engineering 2023, 46(2), 1355-1369. https://doi.org/10.32604/csse.2023.034682
Received 24 July 2022; Accepted 25 November 2022; Issue published 09 February 2023
Abstract
Assistive devices for disabled people with the help of Brain-Computer Interaction (BCI) technology are becoming vital bio-medical engineering. People with physical disabilities need some assistive devices to perform their daily tasks. In these devices, higher latency factors need to be addressed appropriately. Therefore, the main goal of this research is to implement a real-time BCI architecture with minimum latency for command actuation. The proposed architecture is capable to communicate between different modules of the system by adopting an automotive, intelligent data processing and classification approach. Neuro-sky mind wave device has been used to transfer the data to our implemented server for command propulsion. Think-Net Convolutional Neural Network (TN-CNN) architecture has been proposed to recognize the brain signals and classify them into six primary mental states for data classification. Data collection and processing are the responsibility of the central integrated server for system load minimization. Testing of implemented architecture and deep learning model shows excellent results. The proposed system integrity level was the minimum data loss and the accurate commands processing mechanism. The training and testing results are 99% and 93% for custom model implementation based on TN-CNN. The proposed real-time architecture is capable of intelligent data processing unit with fewer errors, and it will benefit assistive devices working on the local server and cloud server.Keywords
Cite This Article
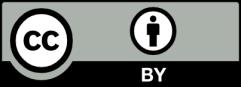
This work is licensed under a Creative Commons Attribution 4.0 International License , which permits unrestricted use, distribution, and reproduction in any medium, provided the original work is properly cited.