Open Access
ARTICLE
A Novel Machine Learning–Based Hand Gesture Recognition Using HCI on IoT Assisted Cloud Platform
1 School of Engineering, Swami Vivekananda University, India
2 Department of Computer Science and Engineering, Sister Nivedita University (Techno India Group) Kolkata, West Bengal, India
3 Sambalpur University, Sambalpur, India
4 Department of Computer Applications, Saveetha College of Liberal Arts and Sciences, SIMATS Deemed to be University, Chennai, India
5 Department of Information Systems, College of Computer and Information Sciences, Jouf University, KSA
6 College of Computer and Information Sciences, Jouf University, Sakaka, 72341, Saudi Arabia
7 School of Computer Science (SCS), Taylor’s University, Subang Jaya, 47500, Malaysia
* Corresponding Author: N. Z. Jhanjhi. Email:
Computer Systems Science and Engineering 2023, 46(2), 2123-2140. https://doi.org/10.32604/csse.2023.034431
Received 16 July 2022; Accepted 23 November 2022; Issue published 09 February 2023
Abstract
Machine learning is a technique for analyzing data that aids the construction of mathematical models. Because of the growth of the Internet of Things (IoT) and wearable sensor devices, gesture interfaces are becoming a more natural and expedient human-machine interaction method. This type of artificial intelligence that requires minimal or no direct human intervention in decision-making is predicated on the ability of intelligent systems to self-train and detect patterns. The rise of touch-free applications and the number of deaf people have increased the significance of hand gesture recognition. Potential applications of hand gesture recognition research span from online gaming to surgical robotics. The location of the hands, the alignment of the fingers, and the hand-to-body posture are the fundamental components of hierarchical emotions in gestures. Linguistic gestures may be difficult to distinguish from nonsensical motions in the field of gesture recognition. Linguistic gestures may be difficult to distinguish from nonsensical motions in the field of gesture recognition. In this scenario, it may be difficult to overcome segmentation uncertainty caused by accidental hand motions or trembling. When a user performs the same dynamic gesture, the hand shapes and speeds of each user, as well as those often generated by the same user, vary. A machine-learning-based Gesture Recognition Framework (ML-GRF) for recognizing the beginning and end of a gesture sequence in a continuous stream of data is suggested to solve the problem of distinguishing between meaningful dynamic gestures and scattered generation. We have recommended using a similarity matching-based gesture classification approach to reduce the overall computing cost associated with identifying actions, and we have shown how an efficient feature extraction method can be used to reduce the thousands of single gesture information to four binary digit gesture codes. The findings from the simulation support the accuracy, precision, gesture recognition, sensitivity, and efficiency rates. The Machine Learning-based Gesture Recognition Framework (ML-GRF) had an accuracy rate of 98.97%, a precision rate of 97.65%, a gesture recognition rate of 98.04%, a sensitivity rate of 96.99%, and an efficiency rate of 95.12%.Keywords
Cite This Article
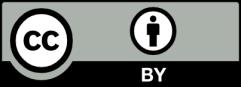
This work is licensed under a Creative Commons Attribution 4.0 International License , which permits unrestricted use, distribution, and reproduction in any medium, provided the original work is properly cited.