Open Access
ARTICLE
Advance IoT Intelligent Healthcare System for Lung Disease Classification Using Ensemble Techniques
1 Department of Networking communications, Faculty of Engineering and Technology, SRM Institute of Science and Technology, Kattankulathur, 603203, Tamilnadu, India
2 Department of Computing Technologies, Faculty of Engineering and Technology, SRM Institute of Science and Technology, Kattankulathur, 603203, Tamilnadu, India
* Corresponding Authors: Prabakaran. Email: ,
Computer Systems Science and Engineering 2023, 46(2), 2141-2157. https://doi.org/10.32604/csse.2023.034210
Received 09 July 2022; Accepted 25 November 2022; Issue published 09 February 2023
Abstract
In healthcare systems, the Internet of Things (IoT) innovation and development approached new ways to evaluate patient data. A cloud-based platform tends to process data generated by IoT medical devices instead of high storage, and computational hardware. In this paper, an intelligent healthcare system has been proposed for the prediction and severity analysis of lung disease from chest computer tomography (CT) images of patients with pneumonia, Covid-19, tuberculosis (TB), and cancer. Firstly, the CT images are captured and transmitted to the fog node through IoT devices. In the fog node, the image gets modified into a convenient and efficient format for further processing. advanced encryption Standard (AES) algorithm serves a substantial role in IoT and fog nodes for preventing data from being accessed by other operating systems. Finally, the preprocessed image can be classified automatically in the cloud by using various transfer and ensemble learning models. Herein different pre-trained deep learning architectures (Inception-ResNet-v2, VGG-19, ResNet-50) used transfer learning is adopted for feature extraction. The softmax of heterogeneous base classifiers assists to make individual predictions. As a meta-classifier, the ensemble approach is employed to obtain final optimal results. Disease predicted image is consigned to the recurrent neural network with long short-term memory (RNN-LSTM) for severity analysis, and the patient is directed to seek therapy based on the outcome. The proposed method achieved 98.6% accuracy, 0.978 precision, 0.982 recalls, and 0.974 F1-score on five class classifications. The experimental findings reveal that the proposed framework assists medical experts with lung disease screening and provides a valuable second perspective.Keywords
Cite This Article
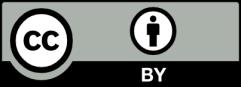
This work is licensed under a Creative Commons Attribution 4.0 International License , which permits unrestricted use, distribution, and reproduction in any medium, provided the original work is properly cited.