Open Access
ARTICLE
Modified Garden Balsan Optimization Based Machine Learning for Intrusion Detection
1 Department of Computer Science, College of Sciences and Humanities-Aflaj, Prince Sattam bin Abdulaziz University, Al-Kharj, Saudi Arabia
2 Department of Industrial Engineering, College of Engineering at Alqunfudah, Umm Al-Qura University, Al Qunfidhah, Saudi Arabia
3 Department of Information Systems, College of Computer and Information Sciences, Princess Nourah bint Abdulrahman University, P.O. Box 84428, Riyadh, 11671, Saudi Arabia
4 Department of Information Technology, College of Computers and Information Technology, Taif University, P.O. Box 11099, Taif, 21944, Saudi Arabia
5 Department of Computer and Self Development, Preparatory Year Deanship, Prince Sattam bin Abdulaziz University, AlKharj, Saudi Arabia
* Corresponding Author: Mesfer Al Duhayyim. Email:
Computer Systems Science and Engineering 2023, 46(2), 1471-1485. https://doi.org/10.32604/csse.2023.034137
Received 07 July 2022; Accepted 22 November 2022; Issue published 09 February 2023
Abstract
The Internet of Things (IoT) environment plays a crucial role in the design of smart environments. Security and privacy are the major challenging problems that exist in the design of IoT-enabled real-time environments. Security susceptibilities in IoT-based systems pose security threats which affect smart environment applications. Intrusion detection systems (IDS) can be used for IoT environments to mitigate IoT-related security attacks which use few security vulnerabilities. This paper introduces a modified garden balsan optimization-based machine learning model for intrusion detection (MGBO-MLID) in the IoT cloud environment. The presented MGBO-MLID technique focuses on the identification and classification of intrusions in the IoT cloud atmosphere. Initially, the presented MGBO-MLID model applies min-max normalization that can be utilized for scaling the features in a uniform format. In addition, the MGBO-MLID model exploits the MGBO algorithm to choose the optimal subset of features. Moreover, the attention-based bidirectional long short-term (ABiLSTM) method can be utilized for the detection and classification of intrusions. At the final level, the Aquila optimization (AO) algorithm is applied as a hyperparameter optimizer to fine-tune the ABiLSTM methods. The experimental validation of the MGBO-MLID method is tested using a benchmark dataset. The extensive comparative study reported the betterment of the MGBO-MLID algorithm over recent approaches.Keywords
Cite This Article
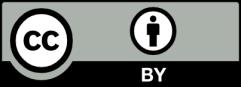
This work is licensed under a Creative Commons Attribution 4.0 International License , which permits unrestricted use, distribution, and reproduction in any medium, provided the original work is properly cited.