Open Access
ARTICLE
Brain Tumor Identification Using Data Augmentation and Transfer Learning Approach
1 Department of Electronics and Communication Engineering, Kongu Engineering College, 638060, Tamil Nadu, India
2 Department of Electronics and Communication Engineering, Sona College of Technology, 636005, Tamil Nadu, India
3 Department of Computer Science and Engineering, Sri Sairam Institute of Technology, Chennai, 600044, Tamil Nadu, India
4 Department of Electrical and Electronics Engineering, Sri Sairam Institute of Technology, Chennai, 600044, Tamil Nadu, India
5 Department of Computer Science and Engineering, Sri Venkateswara College of Engineering, Sriperumbudur, 602117, Tamil Nadu, India
6 Department of Electrical and Electronics Engineering, Sri Venkateswara College of Engineering, Sriperumbudur, 602117, Tamil Nadu, India
7 Department of Computer Science, College of Computing, Khon Kaen University, KhonKaen, 40002, Thailand
8 School of Telecommunication Engineering, Suranaree University of Technology, Nakhon Ratchasima, 30000, Thailand
9 Department of Computer Science, College of Computer and Information Sciences, Majmaah University, Al-Majmaah, 11952, Saudi Arabia
10 Department of Computer Engineering, College of Computer and Information Sciences, Majmaah University, Al-Majmaah, 11952, Saudi Arabia
* Corresponding Author: Chitapong Wechtaisong. Email:
Computer Systems Science and Engineering 2023, 46(2), 1845-1861. https://doi.org/10.32604/csse.2023.033927
Received 01 July 2022; Accepted 04 November 2022; Issue published 09 February 2023
Abstract
A brain tumor is a lethal neurological disease that affects the average performance of the brain and can be fatal. In India, around 15 million cases are diagnosed yearly. To mitigate the seriousness of the tumor it is essential to diagnose at the beginning. Notwithstanding, the manual evaluation process utilizing Magnetic Resonance Imaging (MRI) causes a few worries, remarkably inefficient and inaccurate brain tumor diagnoses. Similarly, the examination process of brain tumors is intricate as they display high unbalance in nature like shape, size, appearance, and location. Therefore, a precise and expeditious prognosis of brain tumors is essential for implementing the of an implicit treatment. Several computer models adapted to diagnose the tumor, but the accuracy of the model needs to be tested. Considering all the above mentioned things, this work aims to identify the best classification system by considering the prediction accuracy out of AlexNet, ResNet 50, and Inception V3. Data augmentation is performed on the database and fed into the three convolutions neural network (CNN) models. A comparison line is drawn between the three models based on accuracy and performance. An accuracy of 96.2% is obtained for AlexNet with augmentation and performed better than ResNet 50 and Inception V3 for the 120th epoch. With the suggested model with higher accuracy, it is highly reliable if brain tumors are diagnosed with available datasets.Keywords
Cite This Article
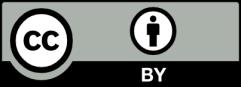
This work is licensed under a Creative Commons Attribution 4.0 International License , which permits unrestricted use, distribution, and reproduction in any medium, provided the original work is properly cited.