Open Access
ARTICLE
Liver Tumor Decision Support System on Human Magnetic Resonance Images: A Comparative Study
1 Department of Biomedical Systems and Informatics Engineering, Yarmouk University 556, Irbid, 21163, Jordan
2 Department of Biomedical Engineering, Jordan University of Science and Technology, Irbid, 22110, Jordan
3 Department of Computer Engineering, Yarmouk University 556, Irbid, 21163, Jordan
4 The of Biomedical Technology, King Hussein Medical Center, Royal Jordanian Medical Service, Amman, 11855, Jordan
5 Faculty of Electrical Engineering & Technology, Campus Pauh Putra, Universiti Malaysia Perlis, Arau, 02600, Perlis, Malaysia
6 Faculty of Electronic Engineering & Technology, Campus Pauh Putra, Universiti Malaysia Perlis, Arau, 02600, Perlis, Malaysia
7 Advanced Computing, Centre of Excellence (CoE), Universiti Malaysia Perlis, Arau, 02600, Perlis, Malaysia
* Corresponding Author: Wan Azani Mustafa. Email:
Computer Systems Science and Engineering 2023, 46(2), 1653-1671. https://doi.org/10.32604/csse.2023.033861
Received 29 June 2022; Accepted 19 October 2022; Issue published 09 February 2023
Abstract
Liver cancer is the second leading cause of cancer death worldwide. Early tumor detection may help identify suitable treatment and increase the survival rate. Medical imaging is a non-invasive tool that can help uncover abnormalities in human organs. Magnetic Resonance Imaging (MRI), in particular, uses magnetic fields and radio waves to differentiate internal human organs tissue. However, the interpretation of medical images requires the subjective expertise of a radiologist and oncologist. Thus, building an automated diagnosis computer-based system can help specialists reduce incorrect diagnoses. This paper proposes a hybrid automated system to compare the performance of 3D features and 2D features in classifying magnetic resonance liver tumor images. This paper proposed two models; the first one employed the 3D features while the second exploited the 2D features. The first system uses 3D texture attributes, 3D shape features, and 3D graphical deep descriptors beside an ensemble classifier to differentiate between four 3D tumor categories. On top of that, the proposed method is applied to 2D slices for comparison purposes. The proposed approach attained 100% accuracy in discriminating between all types of tumors, 100% Area Under the Curve (AUC), 100% sensitivity, and 100% specificity and precision as well in 3D liver tumors. On the other hand, the performance is lower in 2D classification. The maximum accuracy reached 96.4% for two classes and 92.1% for four classes. The top-class performance of the proposed system can be attributed to the exploitation of various types of feature selection methods besides utilizing the ReliefF features selection technique to choose the most relevant features associated with different classes. The novelty of this work appeared in building a highly accurate system under specific circumstances without any processing for the images and human input, besides comparing the performance between 2D and 3D classification. In the future, the presented work can be extended to be used in the huge dataset. Then, it can be a reliable, efficient Computer Aided Diagnosis (CAD) system employed in hospitals in rural areas.Keywords
Cite This Article
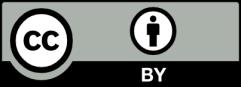