Open Access
ARTICLE
Dragonfly Optimization with Deep Learning Enabled Sentiment Analysis for Arabic Tweets
College of Computer Science and Information Systems, Najran University, Najran, 61441, Saudi Arabia
* Corresponding Author: Hanan T. Halawani. Email:
Computer Systems Science and Engineering 2023, 46(2), 2555-2570. https://doi.org/10.32604/csse.2023.031246
Received 13 April 2022; Accepted 16 June 2022; Issue published 09 February 2023
Abstract
Sentiment Analysis (SA) is one of the Machine Learning (ML) techniques that has been investigated by several researchers in recent years, especially due to the evolution of novel data collection methods focused on social media. In literature, it has been reported that SA data is created for English language in excess of any other language. It is challenging to perform SA for Arabic Twitter data owing to informal nature and rich morphology of Arabic language. An earlier study conducted upon SA for Arabic Twitter focused mostly on automatic extraction of the features from the text. Neural word embedding has been employed in literature, since it is less labor-intensive than automatic feature engineering. By ignoring the context of sentiment, most of the word-embedding models follow syntactic data of words. The current study presents a new Dragonfly Optimization with Deep Learning Enabled Sentiment Analysis for Arabic Tweets (DFODL-SAAT) model. The aim of the presented DFODL-SAAT model is to distinguish the sentiments from opinions that are tweeted in Arabic language. At first, data cleaning and pre-processing steps are performed to convert the input tweets into a useful format. In addition, TF-IDF model is exploited as a feature extractor to generate the feature vectors. Besides, Attention-based Bidirectional Long Short Term Memory (ABLSTM) technique is applied for identification and classification of sentiments. At last, the hyperparameters of ABLSTM model are optimized using DFO algorithm. The performance of the proposed DFODL-SAAT model was validated using the benchmark dataset and the outcomes were investigated under different aspects. The experimental outcomes highlight the superiority of DFODL-SAAT model over recent approaches.Keywords
Cite This Article
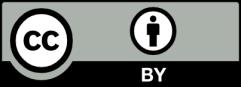