Open Access
ARTICLE
Discharge Summaries Based Sentiment Detection Using Multi-Head Attention and CNN-BiGRU
School of Computer Science and Engineering, Northwestern Polytechnical University, Xi’an, 710072, China
* Corresponding Author: Samer Abdulateef Waheeb. Email:
Computer Systems Science and Engineering 2023, 46(1), 981-998. https://doi.org/10.32604/csse.2023.035753
Received 02 September 2022; Accepted 13 November 2022; Issue published 20 January 2023
Abstract
Automatic extraction of the patient’s health information from the unstructured data concerning the discharge summary remains challenging. Discharge summary related documents contain various aspects of the patient health condition to examine the quality of treatment and thereby help improve decision-making in the medical field. Using a sentiment dictionary and feature engineering, the researchers primarily mine semantic text features. However, choosing and designing features requires a lot of manpower. The proposed approach is an unsupervised deep learning model that learns a set of clusters embedded in the latent space. A composite model including Active Learning (AL), Convolutional Neural Network (CNN), BiGRU, and Multi-Attention, called ACBMA in this research, is designed to measure the quality of treatment based on discharge summaries text sentiment detection. CNN is utilized for extracting the set of local features of text vectors. Then BiGRU network was utilized to extract the text’s global features to solve the issues that a single CNN cannot obtain global semantic information and the traditional Recurrent Neural Network (RNN) gradient disappearance. Experiments prove that the ACBMA method can demonstrate the effectiveness of the suggested method, achieve comparable results to state-of-arts methods in sentiment detection, and outperform them with accurate benchmarks. Finally, several algorithm studies ultimately determined that the ACBMA method is more precise for discharge summaries sentiment analysis.Keywords
Cite This Article
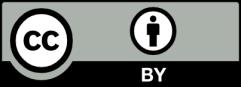
This work is licensed under a Creative Commons Attribution 4.0 International License , which permits unrestricted use, distribution, and reproduction in any medium, provided the original work is properly cited.