Open Access
ARTICLE
An Efficient Automated Technique for Classification of Breast Cancer Using Deep Ensemble Model
1 Department of Electrical Engineering, HITEC University, Taxila, 47080, Pakistan
2 School of Computing, Edinburgh Napier University, Edinburgh, EH10 5DT, UK
3 Department of Computer Science, Faculty of Computing and Information Technology, King Abdulaziz University, Jeddah, 21589, Saudi Arabia
4 Department of Information Technology, College of Computers and Information Technology, Taif University, Taif, 21944, Saudi Arabia
5 DAAI Research Group, Department of Computing and Data Science, School of Computing and Digital Technology, Birmingham City University, 15 Bartholomew Row, Birmingham, B5 5JU, UK
* Corresponding Author: Jawad Ahmad. Email:
Computer Systems Science and Engineering 2023, 46(1), 897-911. https://doi.org/10.32604/csse.2023.035382
Received 18 September 2022; Accepted 15 November 2022; Issue published 20 January 2023
Abstract
Breast cancer is one of the leading cancers among women. It has the second-highest mortality rate in women after lung cancer. Timely detection, especially in the early stages, can help increase survival rates. However, manual diagnosis of breast cancer is a tedious and time-consuming process, and the accuracy of detection is reliant on the quality of the images and the radiologist’s experience. However, computer-aided medical diagnosis has recently shown promising results, leading to the need to develop an efficient system that can aid radiologists in diagnosing breast cancer in its early stages. The research presented in this paper is focused on the multi-class classification of breast cancer. The deep transfer learning approach has been utilized to train the deep learning models, and a pre-processing technique has been used to improve the quality of the ultrasound dataset. The proposed technique utilizes two deep learning models, MobileNetV2 and DenseNet201, for the composition of the deep ensemble model. Deep learning models are fine-tuned along with hyperparameter tuning to achieve better results. Subsequently, entropy-based feature selection is used. Breast cancer identification using the proposed classification approach was found to attain an accuracy of 97.04%, while the sensitivity and F1 score were 96.87% and 96.76%, respectively. The performance of the proposed model is very effective and outperforms other state-of-the-art techniques presented in the literature.Keywords
Cite This Article
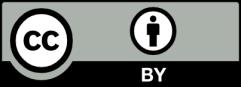
This work is licensed under a Creative Commons Attribution 4.0 International License , which permits unrestricted use, distribution, and reproduction in any medium, provided the original work is properly cited.