Open Access
ARTICLE
MDEV Model: A Novel Ensemble-Based Transfer Learning Approach for Pneumonia Classification Using CXR Images
1 Department of Software Engineering, Mehran University of Engineering and Technology, Jamshoro, Pakistan
2 College of Software, Sungkyunkwan University, Suwon, Korea
3 Department of Computer Systems, Mehran University of Engineering and Technology, Jamshoro, Pakistan
4 Department of Computer Education, Sungkyunkwan University, Seoul, Korea
* Corresponding Authors: Jahwan Koo. Email: ; Nawab Muhammad Faseeh Qureshi. Email:
Computer Systems Science and Engineering 2023, 46(1), 287-302. https://doi.org/10.32604/csse.2023.035311
Received 16 August 2022; Accepted 10 October 2022; Issue published 20 January 2023
Abstract
Pneumonia is a dangerous respiratory disease due to which breathing becomes incredibly difficult and painful; thus, catching it early is crucial. Medical physicians’ time is limited in outdoor situations due to many patients; therefore, automated systems can be a rescue. The input images from the X-ray equipment are also highly unpredictable due to variances in radiologists’ experience. Therefore, radiologists require an automated system that can swiftly and accurately detect pneumonic lungs from chest x-rays. In medical classifications, deep convolution neural networks are commonly used. This research aims to use deep pre-trained transfer learning models to accurately categorize CXR images into binary classes, i.e., Normal and Pneumonia. The MDEV is a proposed novel ensemble approach that concatenates four heterogeneous transfer learning models: MobileNet, DenseNet-201, EfficientNet-B0, and VGG-16, which have been finetuned and trained on 5,856 CXR images. The evaluation matrices used in this research to contrast different deep transfer learning architectures include precision, accuracy, recall, AUC-roc, and f1-score. The model effectively decreases training loss while increasing accuracy. The findings conclude that the proposed MDEV model outperformed cutting-edge deep transfer learning models and obtains an overall precision of 92.26%, an accuracy of 92.15%, a recall of 90.90%, an auc-roc score of 90.9%, and f-score of 91.49% with minimal data pre-processing, data augmentation, finetuning and hyperparameter adjustment in classifying Normal and Pneumonia chests.Keywords
Cite This Article
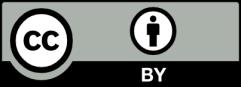
This work is licensed under a Creative Commons Attribution 4.0 International License , which permits unrestricted use, distribution, and reproduction in any medium, provided the original work is properly cited.