Open Access
ARTICLE
LuNet-LightGBM: An Effective Hybrid Approach for Lesion Segmentation and DR Grading
1 School of Computer Science and Engineering, VIT-AP University, Amaravati, Andhra Pradesh, 522237, India
2 School of Computer Science and Engineering, VIT-AP University, Amaravathi, 522237, India
* Corresponding Author: J. Harikiran. Email:
Computer Systems Science and Engineering 2023, 46(1), 597-617. https://doi.org/10.32604/csse.2023.034998
Received 03 August 2022; Accepted 19 October 2022; Issue published 20 January 2023
Abstract
Diabetes problems can lead to an eye disease called Diabetic Retinopathy (DR), which permanently damages the blood vessels in the retina. If not treated early, DR becomes a significant reason for blindness. To identify the DR and determine the stages, medical tests are very labor-intensive, expensive, and time-consuming. To address the issue, a hybrid deep and machine learning technique-based autonomous diagnostic system is provided in this paper. Our proposal is based on lesion segmentation of the fundus images based on the LuNet network. Then a Refined Attention Pyramid Network (RAPNet) is used for extracting global and local features. To increase the performance of the classifier, the unique features are selected from the extracted feature set using Aquila Optimizer (AO) algorithm. Finally, the LightGBM model is applied to classify the input image based on the severity. Several investigations have been done to analyze the performance of the proposed framework on three publically available datasets (MESSIDOR, APTOS, and IDRiD) using several performance metrics such as accuracy, precision, recall, and f1-score. The proposed classifier achieves 99.29%, 99.35%, and 99.31% accuracy for these three datasets respectively. The outcomes of the experiments demonstrate that the suggested technique is effective for disease identification and reliable DR grading.Keywords
Cite This Article
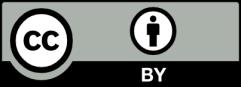