Open Access
ARTICLE
Short-Term Mosques Load Forecast Using Machine Learning and Meteorological Data
Department of Electrical Engineering, College of Engineering, Qassim University, Buraidah, Saudi Arabia
* Corresponding Author: Musaed Alrashidi. Email:
Computer Systems Science and Engineering 2023, 46(1), 371-387. https://doi.org/10.32604/csse.2023.034739
Received 26 July 2022; Accepted 24 October 2022; Issue published 20 January 2023
Abstract
The tendency toward achieving more sustainable and green buildings turned several passive buildings into more dynamic ones. Mosques are the type of buildings that have a unique energy usage pattern. Nevertheless, these types of buildings have minimal consideration in the ongoing energy efficiency applications. This is due to the unpredictability in the electrical consumption of the mosques affecting the stability of the distribution networks. Therefore, this study addresses this issue by developing a framework for a short-term electricity load forecast for a mosque load located in Riyadh, Saudi Arabia. In this study, and by harvesting the load consumption of the mosque and meteorological datasets, the performance of four forecasting algorithms is investigated, namely Artificial Neural Network and Support Vector Regression (SVR) based on three kernel functions: Radial Basis (RB), Polynomial, and Linear. In addition, this research work examines the impact of 13 different combinations of input attributes since selecting the optimal features has a major influence on yielding precise forecasting outcomes. For the mosque load, the (SVR-RB) with eleven features appeared to be the best forecasting model with the lowest forecasting errors metrics giving RMSE, nRMSE, MAE, and nMAE values of 4.207 kW, 2.522%, 2.938 kW, and 1.761%, respectivelyKeywords
Cite This Article
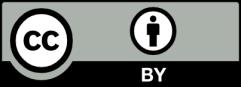
This work is licensed under a Creative Commons Attribution 4.0 International License , which permits unrestricted use, distribution, and reproduction in any medium, provided the original work is properly cited.