Open Access
ARTICLE
Anomaly Detection Based on Discrete Wavelet Transformation for Insider Threat Classification
1 Department of Computer Engineering, Gachon University, Seongnam-si, 13120, Korea
2 Department of AI Software, Gachon University, Seongnam-si, 13120, Korea
* Corresponding Author: Myung-Mook Han. Email:
Computer Systems Science and Engineering 2023, 46(1), 153-164. https://doi.org/10.32604/csse.2023.034589
Received 21 July 2022; Accepted 30 September 2022; Issue published 20 January 2023
Abstract
Unlike external attacks, insider threats arise from legitimate users who belong to the organization. These individuals may be a potential threat for hostile behavior depending on their motives. For insider detection, many intrusion detection systems learn and prevent known scenarios, but because malicious behavior has similar patterns to normal behavior, in reality, these systems can be evaded. Furthermore, because insider threats share a feature space similar to normal behavior, identifying them by detecting anomalies has limitations. This study proposes an improved anomaly detection methodology for insider threats that occur in cybersecurity in which a discrete wavelet transformation technique is applied to classify normal vs. malicious users. The discrete wavelet transformation technique easily discovers new patterns or decomposes synthesized data, making it possible to distinguish between shared characteristics. To verify the efficacy of the proposed methodology, experiments were conducted in which normal users and malicious users were classified based on insider threat scenarios provided in Carnegie Mellon University’s Computer Emergency Response Team (CERT) dataset. The experimental results indicate that the proposed methodology with discrete wavelet transformation reduced the false-positive rate by 82% to 98% compared to the case with no wavelet applied. Thus, the proposed methodology has high potential for application to similar feature spaces.Keywords
Cite This Article
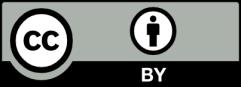