Open Access
ARTICLE
Hyperspectral Images-Based Crop Classification Scheme for Agricultural Remote Sensing
1 Department of Mechanical Engineering, National Taiwan University of Science and Technology, Taipei City, 106335, Taiwan
2 Department of Electrical Engineering, Riphah International University, Islamabad, 44000, Pakistan
3 Department of Mechanical Engineering, HITEC University Taxila, Taxila Cantt., 47080, Pakistan
4 Department of Information Technology, College of Computer and Information Sciences, Princess Nourah bint Abdulrahman University, Riyadh, 11671, Saudi Arabia
5 Department of Electronics and Electrical Communications Engineering, Faculty of Electronic Engineering, Menoufia University, Menouf, 32952, Egypt
6 Security Engineering Laboratory, Department of Computer Science, Prince Sultan University, Riyadh, 11586, Saudi Arabia
* Corresponding Author: Abeer D. Algarni. Email:
Computer Systems Science and Engineering 2023, 46(1), 303-319. https://doi.org/10.32604/csse.2023.034374
Received 15 July 2022; Accepted 30 September 2022; Issue published 20 January 2023
Abstract
Hyperspectral imaging is gaining a significant role in agricultural remote sensing applications. Its data unit is the hyperspectral cube which holds spatial information in two dimensions while spectral band information of each pixel in the third dimension. The classification accuracy of hyperspectral images (HSI) increases significantly by employing both spatial and spectral features. For this work, the data was acquired using an airborne hyperspectral imager system which collected HSI in the visible and near-infrared (VNIR) range of 400 to 1000 nm wavelength within 180 spectral bands. The dataset is collected for nine different crops on agricultural land with a spectral resolution of 3.3 nm wavelength for each pixel. The data was cleaned from geometric distortions and stored with the class labels and annotations of global localization using the inertial navigation system. In this study, a unique pixel-based approach was designed to improve the crops' classification accuracy by using the edge-preserving features (EPF) and principal component analysis (PCA) in conjunction. The preliminary processing generated the high-dimensional EPF stack by applying the edge-preserving filters on acquired HSI. In the second step, this high dimensional stack was treated with the PCA for dimensionality reduction without losing significant spectral information. The resultant feature space (PCA-EPF) demonstrated enhanced class separability for improved crop classification with reduced dimensionality and computational cost. The support vector machines classifier was employed for multiclass classification of target crops using PCA-EPF. The classification performance evaluation was measured in terms of individual class accuracy, overall accuracy, average accuracy, and Cohen kappa factor. The proposed scheme achieved greater than 90 % results for all the performance evaluation metrics. The PCA-EPF proved to be an effective attribute for crop classification using hyperspectral imaging in the VNIR range. The proposed scheme is well-suited for practical applications of crops and landfill estimations using agricultural remote sensing methods.Keywords
Cite This Article
I. Ali, Z. Mushtaq, S. Arif, A. D. Algarni, N. F. Soliman et al., "Hyperspectral images-based crop classification scheme for agricultural remote sensing," Computer Systems Science and Engineering, vol. 46, no.1, pp. 303–319, 2023. https://doi.org/10.32604/csse.2023.034374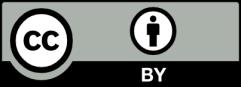