Open Access
ARTICLE
Weight Prediction Using the Hybrid Stacked-LSTM Food Selection Model
1 Faculty of Computers and Information, Computer Science Department, Suez University, Suez, Egypt
2 Faculty of Artificial Intelligence, Kafrelsheikh University, Kafrelsheikh, 33511, Egypt
3 Faculty of Computers and Information, Computer Science Department, Mansoura University, 35561, Egypt
4 Faculty of Physical Education, Track and Field Competitions Department, Arish University, Egypt
5 Delta Higher Institute of Engineering and Technology, Mansoura, 35111, Egypt
6 Department of Computer Science, Arab East Colleges, Riyadh, 13544, Saudi Arabia
* Corresponding Author: Mahmoud Y. Shams. Email:
Computer Systems Science and Engineering 2023, 46(1), 765-781. https://doi.org/10.32604/csse.2023.034324
Received 13 July 2022; Accepted 18 August 2022; Issue published 20 January 2023
Abstract
Food choice motives (i.e., mood, health, natural content, convenience, sensory appeal, price, familiarities, ethical concerns, and weight control) have an important role in transforming the current food system to ensure the healthiness of people and the sustainability of the world. Researchers from several domains have presented several models addressing issues influencing food choice over the years. However, a multidisciplinary approach is required to better understand how various aspects interact with one another during the decision-making procedure. In this paper, four Deep Learning (DL) models and one Machine Learning (ML) model are utilized to predict the weight in pounds based on food choices. The Long Short-Term Memory (LSTM) model, stacked-LSTM model, Conventional Neural Network (CNN) model, and CNN-LSTM model are the used deep learning models. While the applied ML model is the K-Nearest Neighbor (KNN) regressor. The efficiency of the proposed model was determined based on the error rate obtained from the experimental results. The findings indicated that Mean Absolute Error (MAE) is 0.0087, the Mean Square Error (MSE) is 0.00011, the Median Absolute Error (MedAE) is 0.006, the Root Mean Square Error (RMSE) is 0.011, and the Mean Absolute Percentage Error (MAPE) is 21. Therefore, the results demonstrated that the stacked LSTM achieved improved results compared with the LSTM, CNN, CNN-LSTM, and KNN regressor.Keywords
Cite This Article
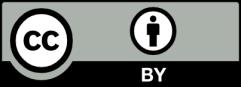
This work is licensed under a Creative Commons Attribution 4.0 International License , which permits unrestricted use, distribution, and reproduction in any medium, provided the original work is properly cited.