Open Access
ARTICLE
A Lightweight Deep Autoencoder Scheme for Cyberattack Detection in the Internet of Things
1 Information Systems Department, Faculty of Computing and Information Technology, King Abdulaziz University, Jeddah, 80200, Saudi Arabia
2 School of Computing, Edinburgh Napier University, Edinburgh EH10 5DY, UK
* Corresponding Author: Jawad Ahmad. Email:
Computer Systems Science and Engineering 2023, 46(1), 57-72. https://doi.org/10.32604/csse.2023.034277
Received 12 July 2022; Accepted 22 September 2022; Issue published 20 January 2023
Abstract
The Internet of things (IoT) is an emerging paradigm that integrates devices and services to collect real-time data from surroundings and process the information at a very high speed to make a decision. Despite several advantages, the resource-constrained and heterogeneous nature of IoT networks makes them a favorite target for cybercriminals. A single successful attempt of network intrusion can compromise the complete IoT network which can lead to unauthorized access to the valuable information of consumers and industries. To overcome the security challenges of IoT networks, this article proposes a lightweight deep autoencoder (DAE) based cyberattack detection framework. The proposed approach learns the normal and anomalous data patterns to identify the various types of network intrusions. The most significant feature of the proposed technique is its lower complexity which is attained by reducing the number of operations. To optimally train the proposed DAE, a range of hyperparameters was determined through extensive experiments that ensure higher attack detection accuracy. The efficacy of the suggested framework is evaluated via two standard and open-source datasets. The proposed DAE achieved the accuracies of 98.86%, and 98.26% for NSL-KDD, 99.32%, and 98.79% for the UNSW-NB15 dataset in binary class and multi-class scenarios. The performance of the suggested attack detection framework is also compared with several state-of-the-art intrusion detection schemes. Experimental outcomes proved the promising performance of the proposed scheme for cyberattack detection in IoT networks.Keywords
Cite This Article
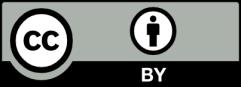