Open Access
ARTICLE
A Hybrid Approach for Plant Disease Detection Using E-GAN and CapsNet
Department of Data Science and Business Systems, School of Computing, SRM Institute of Science and Technology, Kattankulathur, Tamilnadu, 603203, India
* Corresponding Author: N. Vasudevan. Email:
Computer Systems Science and Engineering 2023, 46(1), 337-356. https://doi.org/10.32604/csse.2023.034242
Received 11 July 2022; Accepted 08 October 2022; Issue published 20 January 2023
Abstract
Crop protection is a great obstacle to food safety, with crop diseases being one of the most serious issues. Plant diseases diminish the quality of crop yield. To detect disease spots on grape leaves, deep learning technology might be employed. On the other hand, the precision and efficiency of identification remain issues. The quantity of images of ill leaves taken from plants is often uneven. With an uneven collection and few images, spotting disease is hard. The plant leaves dataset needs to be expanded to detect illness accurately. A novel hybrid technique employing segmentation, augmentation, and a capsule neural network (CapsNet) is used in this paper to tackle these challenges. The proposed method involves three phases. First, a graph-based technique extracts leaf area from a plant image. The second step expands the dataset using an Efficient Generative Adversarial Network E-GAN. Third, a CapsNet identifies the illness and stage. The proposed work has experimented on real-time grape leaf images which are captured using an SD1000 camera and PlantVillage grape leaf datasets. The proposed method achieves an effective classification of accuracy for disease type and disease stages detection compared to other existing models.Keywords
Cite This Article
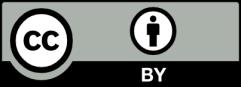
This work is licensed under a Creative Commons Attribution 4.0 International License , which permits unrestricted use, distribution, and reproduction in any medium, provided the original work is properly cited.