Open Access
ARTICLE
3D Object Detection with Attention: Shell-Based Modeling
1 School of Computer and Software, Nanjing University of Information Science & Technology, Nanjing, 210044, China
2 Wuxi Research Institute, Nanjing University of Information Science & Technology, Wuxi, 214100, China
3 Engineering Research Center of Digital Forensics, Ministry of Education, Jiangsu Engineering Center of Network Monitoring, Nanjing, 210044, China
4 Jiangsu Collaborative Innovation Center of Atmospheric Environment and Equipment Technology (CICAEET), Nanjing University of Information Science & Technology, Nanjing, 210044, China
5 School of Automation, Nanjing University of Information Science & Technology, Nanjing, 210044, China
6 Department of Electrical and Computer Engineering, University of Windsor, Windsor, N9B 3P4, Canada
* Corresponding Author: Xiaorui Zhang. Email:
Computer Systems Science and Engineering 2023, 46(1), 537-550. https://doi.org/10.32604/csse.2023.034230
Received 10 July 2022; Accepted 10 October 2022; Issue published 20 January 2023
Abstract
LIDAR point cloud-based 3D object detection aims to sense the surrounding environment by anchoring objects with the Bounding Box (BBox). However, under the three-dimensional space of autonomous driving scenes, the previous object detection methods, due to the pre-processing of the original LIDAR point cloud into voxels or pillars, lose the coordinate information of the original point cloud, slow detection speed, and gain inaccurate bounding box positioning. To address the issues above, this study proposes a new two-stage network structure to extract point cloud features directly by PointNet++, which effectively preserves the original point cloud coordinate information. To improve the detection accuracy, a shell-based modeling method is proposed. It roughly determines which spherical shell the coordinates belong to. Then, the results are refined to ground truth, thereby narrowing the localization range and improving the detection accuracy. To improve the recall of 3D object detection with bounding boxes, this paper designs a self-attention module for 3D object detection with a skip connection structure. Some of these features are highlighted by weighting them on the feature dimensions. After training, it makes the feature weights that are favorable for object detection get larger. Thus, the extracted features are more adapted to the object detection task. Extensive comparison experiments and ablation experiments conducted on the KITTI dataset verify the effectiveness of our proposed method in improving recall and precision.Keywords
Cite This Article
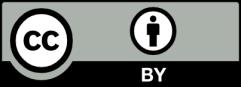
This work is licensed under a Creative Commons Attribution 4.0 International License , which permits unrestricted use, distribution, and reproduction in any medium, provided the original work is properly cited.